What Is a Data Warehouse?
Enterprises large and small are trying to leverage data to enhance their businesses using data analytics. Because large amounts of data are now being generated, businesses have to find a way to store and access this data in an accessible, secure, cost-effective, and efficient way. There are several options for storing data, including databases, data lakes, and cloud storage. This article focuses on the data warehouse as a means of efficient and effective data storage.
A data warehouse can be defined as a central repository that ingests data from different sources, like relational databases and transactional systems. They are designed to store large amounts of data and enable organizations to analyze and present data accurately.
Data warehouses are being used in all kinds of industries, including health care, banking, and retail. For instance, when you store all of your data in a data warehouse, it can be utilized in the retail industry to pull information like customer lifetime value analysis, market basket analysis, customer buying behavior analysis, and more.
In the healthcare industry, once data is stored in a warehouse, it can be used for its analytics capabilities. Visualization and reporting tools can be used to forecast and present results that can predict the health conditions of patients or analyze abnormalities in the health of a patient. Banking services can utilize data warehouses to analyze data and improve their services while generating reports to present to stakeholders.
Data warehouses offer a wide range of benefits, including historical data storage, data security, data consistency, and data stability.
In this article, you’ll learn about the benefits and features of a data warehouse and how to effectively implement, use, and maintain a data warehouse.
Data Warehouse Features and Use Cases
Data warehouses have many features and uses, including the following:
- Built Scalable: Data warehouses are built with seamless scalability in mind. A data warehouse can grow from megabytes to gigabytes to terabytes of data with the push of a button, all without the users or administrators having to worry about replication, sharding, etc.
- Subject oriented: They store historical data, including previous sales, marketing campaigns, and human resources information. This data helps organizations gain insights and make significant predictions to improve business operations.
- Data Engineering Ready: Warehouses have a large ecosystem of data processing tools built on top of them. For example, dbt is a data modeling tool set that is meant to work with data warehouses.
- Integration: They store data from multiple sources and can be synchronized to structure the data.
- Persistence Data is stored in resilient, fault-tolerant ways to prevent data loss.
- Time variant: Because data warehouses store historical data, you can access data based on a timestamp and check if there are changes to the data over time.
- Informed decision-making: Data stored in the warehouse is used by different data professionals to make efficient data-driven decisions.
- Better data visualization: Some warehouses provide different visualization and reporting tools to analyze and present data efficiently. You can also use external visualization tools like Tableau, Power BI, Plotly, QlikView etc. These tools tend to have a wide variety of connectors to securely connect to your warehouse and power their visualizations.
Data warehouses not only store data but also provide value. They consolidate data from different sources and synchronize it in a way that data from each source is formatted similarly. This structured data is then used by data analysts and data scientists to create visualizations and make predictions.
Here are some of the main ways data warehouses can be utilized:
- Customer data: Companies can collect data to use for analytics and machine learning to improve services. Businesses, like Facebook and Google, collect customer data to improve user engagement. Keeping this data secure is vitally important. When using data warehouses as a storage option, you need to choose security features like strict user access control, encryption in data transfer, and other important security mechanisms.
- Machine learning (ML): Data stored in a warehouse can be used for all kinds of analysis and predictions. ML applications can be used for personalization, churn prediction, weather forecasting, self-driving cars, health monitoring, and more.
- Data sanitation: As you add data to a warehouse, it becomes harder to manage. If you keep adding data, you’ll need to continually increase your storage capacity, which can become cost prohibitive. To keep costs in check, you can sanitize your data. Sanitation is the process of deleting data from your warehouses that is no longer useful.
It’s important to note that once data is deleted from the warehouse, you’re unable to retrieve it.
- Real-time access and analysis: Some use cases require real-time access to data. For example, analysis of a patient’s health condition. In this case, a warehouse is useful for accessing data whenever it’s needed.
Data Warehouse Architecture
A data warehouse is made up of three tiers. Each tier helps different data professionals to leverage the data.
- Tier 1: This is considered to be the bottom tier of the warehouse. This layer is usually a relational database that ingests data from different data sources. It’s responsible for holding all the data.
- Tier 2: Tier 2 or the middle tier is where data is accessed and processed. This layer is made up of online analytical processing (OLAP) servers that are used to fetch and analyze data from the warehouse.
- Tier 3: The top tier or Tier 3 is the frontend comprised of various visualization and reporting tools that enable users to analyze and present data to different stakeholders and customers.
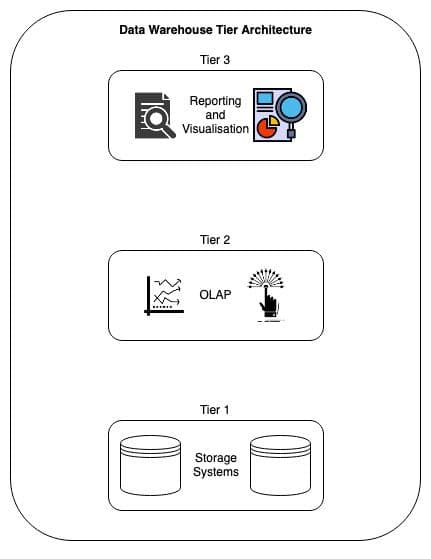
A data warehouse is not just a storage mechanism. You should consider it as a platform that is used for data analysis, data mining, machine learning (ML), and artificial intelligence (AI) for a large amount of historical data.
ETL Process
The ETL (extract, transform, and load) process is an integral part of ingesting data from multiple sources into a data warehouse.
1. Extract: At this stage, data is extracted from various sources, like XML files, relational databases, NoSQL databases, and sensors. Data is then fed to a staging area before it’s fed to the warehouse. The staging area helps format the data so it doesn’t damage the warehouse and make it impossible to roll back.
2. Transform: Data from various sources is transformed to a standard format and fed into the warehouse. The tasks that transform the data include the following:
- Data cleaning: Data fetched from multiple sources may have some missing or corrupted values. In this stage, the data is cleaned to remove such values.
- Data filtering: Filtering data helps you load only specific fields from various sources.
- Data joining: For specific needs, data from various sources are joined together into one.
- Data sorting: In this stage, data is sorted per requirement.
- Data normalizing: ML and analytic algorithms require data to be normalized so that all the attributes are on the same scale.
3. Load: This is the final stage where transformed and cleaned data is fed to the warehouse. Usually, data is ingested as a scheduled batch operation. This can be also be done manually if required.
There are two categories of ETL: Batch ETL and Streaming ETL.
Batch ETL vs Streaming ETL
In the traditional ETL or Batch ETL process, ETL software extracts data from various sources on a scheduled basis, transforms it by applying various transformations, and finally loads it into a warehouse.
On the other hand, the Streaming ETL process moves real-time data from one place to another automatically. Streaming ETL is important for new technologies like IoT, online retailing, and banking services because they generate data in real-time at a great velocity.
Data Warehouse Components
Data warehouses are built, using various components, according to the needs of an organization. These components coordinate with each other to help data specialists make use of the data. They include the following:
- External data sources: These are the sources from where the raw data is fetched to feed the data warehouse.
- Data staging area: A temporary storage space to store data before it is batch loaded into the warehouse. In the case of cloud data warehouses, this often takes the form of AWS S3 or Google Cloud Storage buckets.
- Data Warehouse Database Management System(DBMS): A variation of the traditional DBMS of relational databases. Since data warehouses are often columnar and not row based, these DBMS often provide extra functionality like maintaining sort keys, shard distribution, and more.
- Data marts: Data marts are an optional component that is dependent on the organization’s needs. Warehouses collect all the data for every LoB in an organization while data marts are used to store LoB-specific data that is collected only from the warehouse.
- Data mining tools: This is a collection of tools used for data mining and knowledge discovery.
- Online Analytical Processing Server (OLAP): The OLAP is a collection of various software tools used for analysis and business decisions.
- Reporting tools: These tools are used for data visualization and reporting.
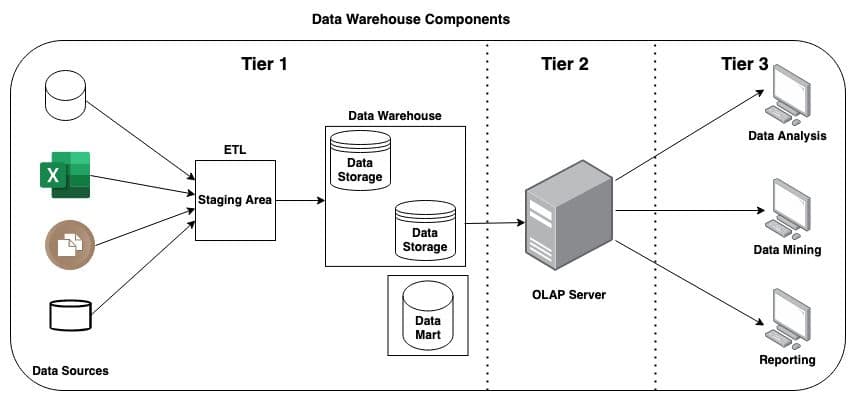
Things to Consider When Designing a Data Warehouse
Both logical and physical designs should be considered when designing a data warehouse.
Logical design refers to the relationship between different entities in the warehouse, whereas the storage and retrieval of data are considered in the physical design.
Before designing a data warehouse, it’s important to consider the following:
- Type of data that will be stored in the warehouse
- Relationship between and within data groups
- Different transformations required for data before storage
- Refresh rate of the data
Existing Options for Running a Data Warehouse
There are currently several existing options for running a data warehouse, including Snowflake, Amazon Redshift, Google BigQuery, and Microsoft Azure, as well as a few other storage services that offer unique advantages.
Snowflake
Snowflake was developed on top of the cloud infrastructures of Amazon Web Services (AWS), Microsoft Azure, and Google. There’s no hardware or software to select, install, configure, or administer; so it’s ideal for businesses that do not want to spend resources on the setup, maintenance, and support of in-house servers.
Redshift
Redshift is a warehouse service provided by Amazon and is a major component of AWS. It’s a fully managed petabyte-scale cloud data warehouse tool for storing and analyzing large data sets. It can also perform large-scale database migrations.
BigQuery
BigQuery is a data warehouse service provided by Google that offers functionality to analyze petabytes of data. BigQuery provides various tools that can be used to access, analyze, and report on the data present in it.
BigQuery is normally used at the end of big data ETL pipelines or for complex analytical queries and processing.
Microsoft Azure Synapse Analytics
Microsoft Azure is a data warehousing service provided by Microsoft that has robust analytics services. Data integration, enterprise data warehousing, and big data analytics are all part of its analytics package.
It’s considered to be faster than BigQuery and Redshift and is preferred by companies that value speed.
Other Storage Services
PostgreSQL and Amazon S3 are not full-fledged data warehouses but offer functionalities that provide unique advantages for data storage.
PostgreSQL
PostgreSQL is a very advanced database that can work as both a transactional database and an efficient, low-cost data warehouse. Because it’s open source, it’s a free service, unlike the previously mentioned pay-as-you-go options.
Unlike other warehouse services providers, PostgreSQL provides minimal data warehouse services, making it a better fit for small-scale businesses.
Alternative Warehouse Options
There are also other alternatives to consider, including data lakes and large-scale databases.
Data lakes can store structured, semi-structured, and unstructured data. Because of this, data professionals, like data analysts, data scientists, and ML engineers, can utilize the data to make informed business decisions. When data professionals need to work on data, like images and text, data lakes are the preferred storage mechanisms. Data lakes can also be used for regular, structured data storage as a very cost effective option, especially when large scale analysis is not a regular business need.
Traditional databases can’t provide analytic services, but they can hold a large amount of data. You can pair them with external analytics tools, like Tableau, Microsoft Power BI, Looker, or Sigma to help make business decisions. On a smaller scale, data from traditional databases might be used to export data to spreadsheet tools like Microsoft Excel and Google Sheets, or to custom Python scripts.
When data storage is the only requirement of a company, traditional databases are the preferred storage mechanisms.
Data Warehouses vs Transactional Databases
The purpose of a transactional database is to store data for transactional processing. It holds data from a single source and focuses on storing real-time streaming data. It’s generally used for continuous read-and-write operations and falls under the category of OLTP systems.
A data warehouse, however, provides functionalities, like storing high-volume data along with performing various analytics and reporting services. In addition to real-time data, warehouses can hold data from multiple sources, including historical data. Data Warehouses are an example of OLAP systems.
Data Warehouses vs Data Lakes
Data lakes can store all kinds of data, including structured, semi-structured, and unstructured data, in its raw format from different sources, like social media, mobile apps, and IoT devices. This data can be used for ML, deep learning, and business intelligence (BI). Their schemas are designed at the time of implementation and are preferred by data scientists due to their flexibility to store all kinds of data.
Data warehouses can only store structured data from multiple sources in the same format. Warehouses can be used for ML and BI tasks, and their schemas are usually designed prior to implementation. Data analysts and business analysts usually prefer this kind of data because it saves time since the data is already normalized and ready to use.
On-Premise vs Cloud Warehouse
When discussing a data warehouse project, on-premise vs cloud warehouse services is an important issue. On-Premise solutions have two major advantages: They are purpose-built for your organization’s needs and they can offer a lot more security features since you are in physical control of your hardware.
These advantages of course have their drawbacks as well. On-Premise solutions present a significantly higher upfront investment in everything from planning time, staffing needs, hardware requirements for both compute and storage, as well as running maintenance costs for both hardware and software updates. Cloud warehouses on the other hand can easily start at a few dollars per hour and can start within minutes.
For those reasons, on-premise solutions are increasingly rare these days and are most often found in industries (financial data, healthcare data) where the need for security or even compliance with local laws outweighs the speed, flexibility, and/or cost advantages that cloud warehouse solutions offer.
Conclusion
Data is increasing at a rapid pace, and technology must evolve to handle its accumulation. Traditional databases can store data but aren’t able to provide the analytics and insights that businesses need to make accurate and perceptive decisions.
Data warehousing is a vital tool for organizations ready to put their data to good use in everything from more robust analytics and dashboarding solutions, to ML applications, future planning, and trend forecasting.
If you are interested in a more practical approach to a data warehouse solution, consider these articles:
- Data Warehouse Architecture discusses use cases such as ETL, ELT, streaming vs batch data, event-stream data vs record data, and more
- Data Warehouses versus Databases discusses how a data warehouse is designed and meant to be used completely differently from a traditional transactional database.
- How to Create and Use Business Intelligence with a Data Warehouse discusses different kinds of business intelligence tooling and how data warehouses support performing BI tasks at scale.
The Data Maturity Guide
Learn how to build on your existing tools and take the next step on your journey.
Build a data pipeline in less than 5 minutes
Create an accountSee RudderStack in action
Get a personalized demoCollaborate with our community of data engineers
Join Slack Community