What is a Data Dictionary?
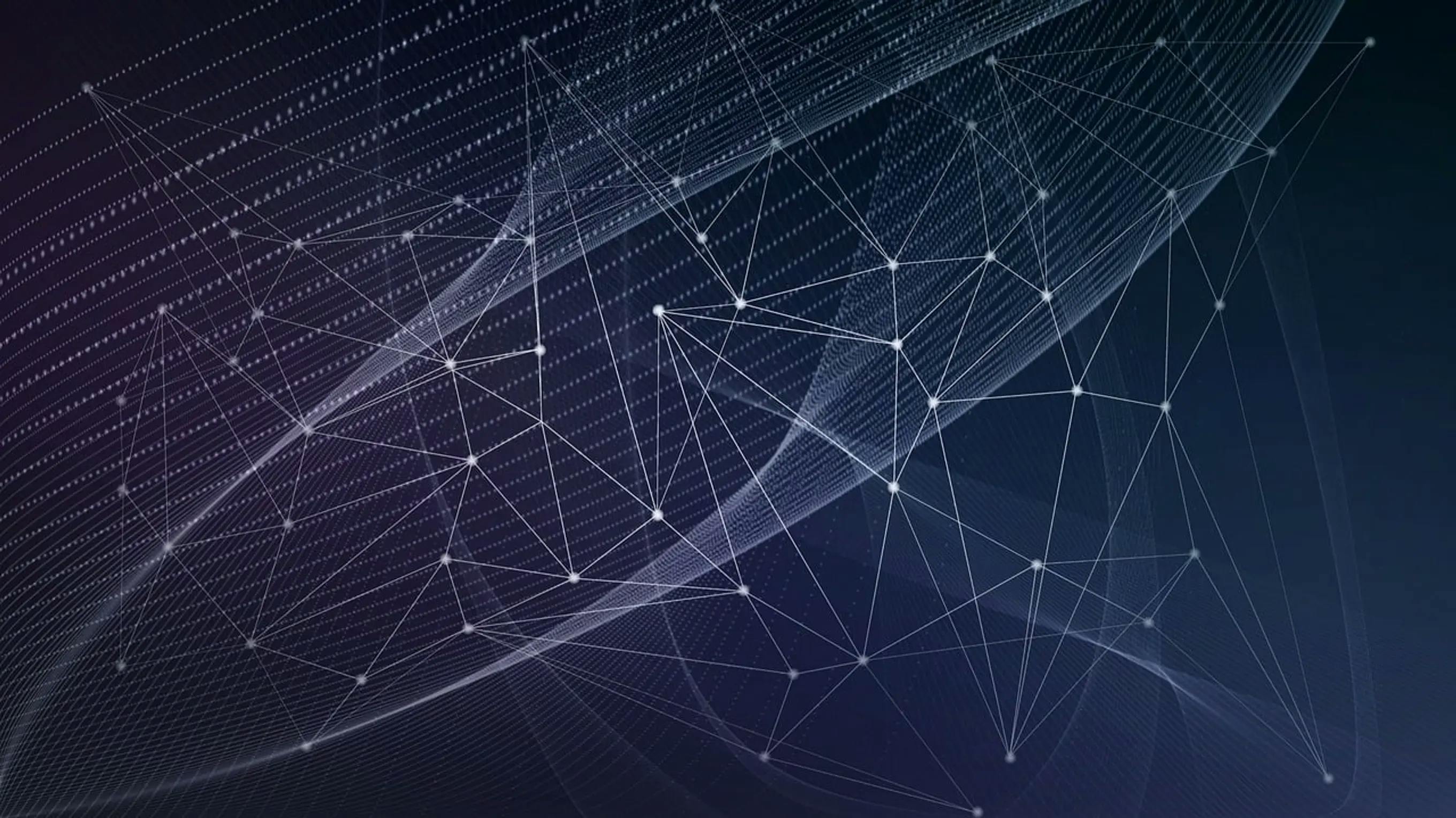
Learn about the Data Dictionary to improve your data governance and quality by providing pertinent context, and fostering transparency among stakeholders.
Figuring out how to evaluate and utilize data is one of the most complex tasks for stakeholders in data management. This is especially true as data elements increase and the necessity for managing information within the information system becomes vital.The crucial element to build a mutual understanding of the vision and utilization of the data is a data dictionary. It serves as the foundation for cooperation among various stakeholders within an information system. It not only stores information about data types and data elements but also plays a vital role in advancing business rules and ensuring data quality. The active data dictionary aids in data analysis and decision-making, thereby enhancing the data integrity within the organization. It acts as a template for database tables, defining field names and default values, making it an indispensable part of database management systems.
What is a Data Dictionary?
A data dictionary serves as a compilation of attributes and data definitions for data elements and field names within a database. The primary role of a data dictionary is to supply your data models with pertinent context, enabling your stakeholders and database administrators to gain a deeper understanding of the fundamental business glossary enclosed in your data warehouse. A data dictionary exists as a standalone document offering detailed metadata and crucial information.
As data models continue to evolve and become more intricate, encompassing numerous data objects and fields, the challenge to comprehend these data definitions escalates. Suppose your information system houses multiple customer terminologies such as LTV, MRR, churn rate, active workspaces, playlists, and so forth. In that case, a data dictionary serves as a handy tool, offering a concise explanation and summary for each of these data elements. The principal role of a data dictionary is to act as a single source of truth for all your business rules. This approach aids in curtailing inefficiencies, enhancing data quality and data integrity, and ensuring alignment between database administrators and business stakeholders. It's also an essential component of effective data management and data governance, ensuring that your database management systems operate optimally.
Three things to consider in Data Dictionary design
Architecting an operative data dictionary can be an intimidating project, especially when dealing with large data types and data assets. Hence, establishing a robust foundation for data management is fundamental to achieve this goal. There are several pivotal queries you must ponder on, such as data integrity, data quality, the role of database administrators, and deciding on the types of data dictionaries to use, whether active data dictionary or passive data dictionary. Also, consider the business rules, how to handle metadata, the significance of data elements in your dataset, and how your database management systems (DBMS) can aid in validation.
- What is the source of the data, and does it uphold data integrity?
- How will different stakeholders and organizations utilize the data in theory and real-world scenarios?
- Which data elements are you looking to leverage, and how can you ascertain their relevance for data analysis?
1. Sources
Data can originate from a multitude of touchpoints such as websites, applications, advertisements, emails, social channels, and more. It's crucial to enhance these data elements with extra context from complementing sources such as your sales cycle. Defining your data source should not be a one-time event. Your primary focus should be on creating a data pipeline that is consistently updated and managed under robust data management practices. In most cases, a data dictionary might not be useful if your data isn't flowing into your database management systems, which serve as your single source of truth, ensuring data integrity. Continuous data compilation and validation help enhance the data quality, essential for decision-making processes.
2. Outputs
Comprehending the structure and interpretation of data by diverse stakeholders scrutinizing this data asset is essential for boosting multi-application. Once these data elements and data types are defined and accessible for use within the information system, data governance becomes a high priority to curtail redundancy and enhance accessibility, promoting efficiency in data management. This understanding is key in the decision-making process.
3. Application
The crucial stage in constructing any valuable data assets involves grasping the use cases and establishing business rules around them. Essentially, your goal should be to form an information system that promotes value and reinforces the theories derived from data analysis and analytics.
How to build a Data Dictionary
Let’s break it down to 7 steps to build a Data Dictionary
1. Understand the terminologies
The initial phase involves gathering a comprehensive data dictionary, which includes all the terminology or jargon utilized in your firm's databases, business glossary, and marketing technology solutions. This can be achieved through active engagement with stakeholders, merging your knowledge from various data sources such as reports, dashboards, metrics, and OKRs.
2. Create definitions
After you have compiled all the essential data elements, utilizing existing data assets such as documentation can help in populating data definitions for fundamental terms and meanings, effectively enhancing data management. This approach can provide valuable insights into which business sectors should receive more resources and which stakeholders can aid others in creating appropriate documentation in the future. It's important to note any duplicate or ambiguous terms within your data dictionary as this will assist in prioritizing during future rounds of data analysis.
3. Highlight conflicting definitions
With all data sources visible and the information system fully disclosed, the next phase involves collectively examining the data elements that seemingly contradict. You must compile a list of these conflicting data definitions and coordinate subsequent meetings with the relevant stakeholders to clarify these definitions. Constructing a scalable data dictionary at this juncture can prevent such meetings down the line.
4. Ensure consistent definitions
Assign a stakeholder, akin to a project manager, to mediate during the process, particularly during instances of disagreement among teams. This individual should handle all feedback and assist in defining data definitions to ensure consistency and data integrity. This role is akin to a database administrator in a DBMS (Database Management Systems), ensuring the system adheres to business rules and maintaining the active data dictionary. The goal is to establish a consistent data model and enhance data quality while promoting effective decision-making.
5. Get approval
Ensure that stakeholders and higher management have endorsed your data management strategy and are cognizant of it. Without their consent, maintaining this novel approach could become a hurdle, and there's a risk of teams creating their own data dictionaries. This could lead to a scenario where database management systems are filled with multiple types of data dictionaries, jeopardizing data integrity and leading you back to square one.
6. Publish
After finalizing your data definitions, the concluding stage involves publishing your data dictionary. Keep these in a centralized repository where it is accessible to all the stakeholders.
7. Optimize
The data dictionary should be considered a dynamic, active data dictionary that continuously evolves as your stakeholders introduce new data elements and data definitions. As part of effective data management, it's crucial to establish a protocol for implementing these upcoming modifications across different teams. This involves designating one team with the authority to validate a definition, another to actualize the change in the database management systems, and a third to comprehend its impact on reports and metrics. This approach ensures data integrity and contributes to data governance, enhancing the overall data quality within the information system.
Using a Warehouse Native CDP can help make this process more reliable and easy to maintain.
Examples and resources
- United States Geological Survey website provides ample resources and examples for the topic of Data Dictionary
- Northwest Environment Data published a report on best practices to use their data dictionary and definitions
Benefits
Data dictionaries offer numerous benefits, but their core advantage lies in fostering transparency and creating explicit documentation for cross-functional teams engaged in data management. By employing data dictionaries, it's possible to enhance data navigation and refine data search, two critical elements in effective database management systems. Moreover, they are instrumental in pinpointing anomalies across varying projects and teams in the information system. They effectively help in eliminating superfluous and duplicative data elements, thereby improving data quality and ensuring data integrity. This increases confidence in your data and the database integrity.
Final Thoughts
In an era where data management and database management systems are pivotal, it is vital to realize the influence of today's dynamic work environments. Now, the triumph of any entity is heavily reliant on nurturing productive and enduring collaboration within and between teams, rather than their technical advancements. This collaboration involves various stakeholders and utilizing data assets such as data dictionaries, metadata, data elements, and data types.
In the context of marketing teams, the ambiguous terrain of technical, creative, brand, and retention marketing roles can pose unknown challenges to businesses. Ultimately, this demands that all team sections function with a consolidated comprehension of key data definitions and metrics that drive success through strategic decision-making. The absence of proper documentation can cause confusion and miscommunication among marketers harnessing data elements for personalized marketing and first-party data audience engagement. This can ultimately lead to a rift in team coordination and the overall business strategy. The remedy lies in organizing an active data dictionary, serving as a single data source of truth. This not only empowers cross-functional relationships but also promotes data-driven collaboration and strategies, reinforcing effective data management within the team.
Recent Posts
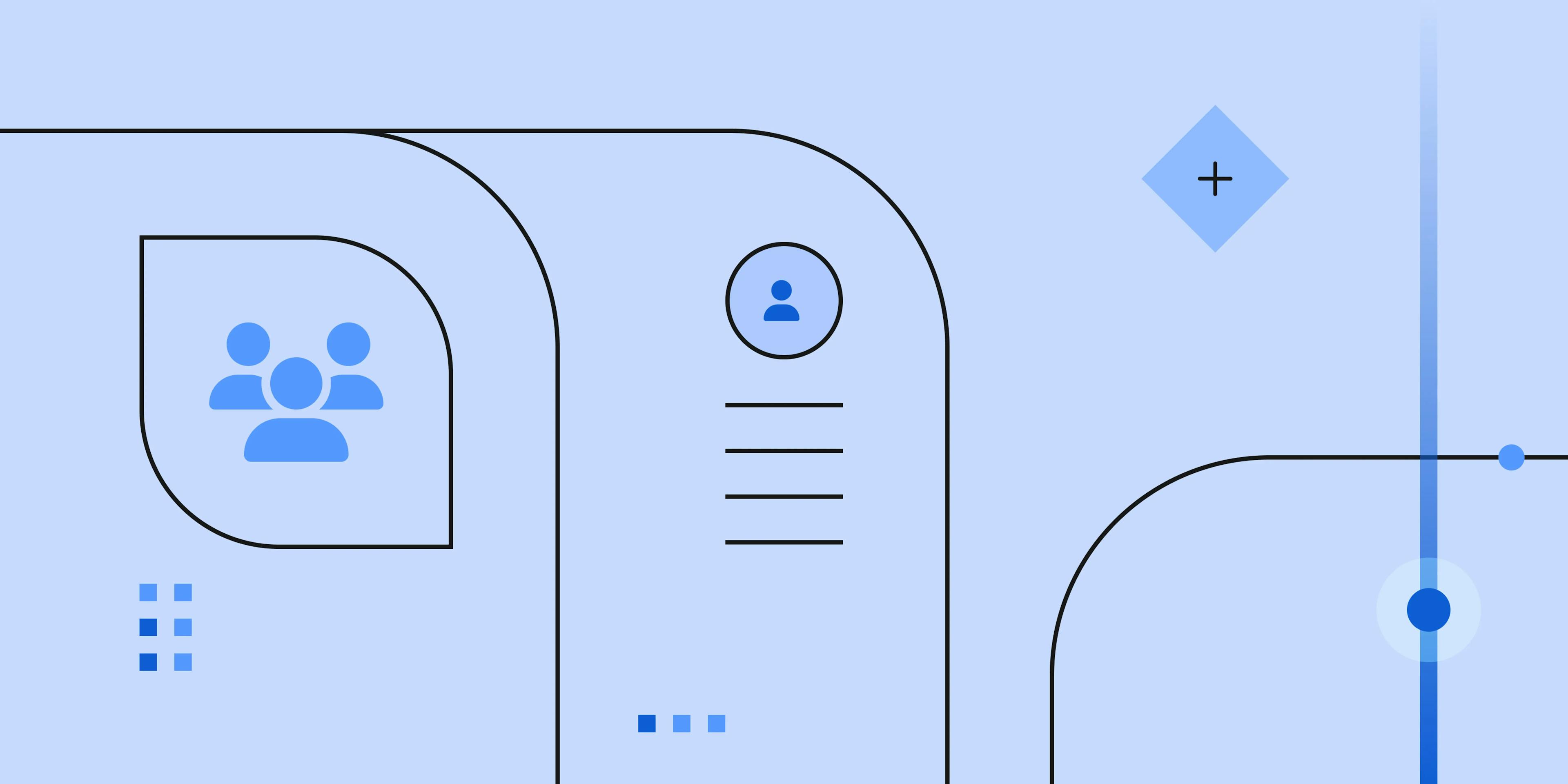
Why Shopify will increasingly require data engineering expertise
By John Wessel
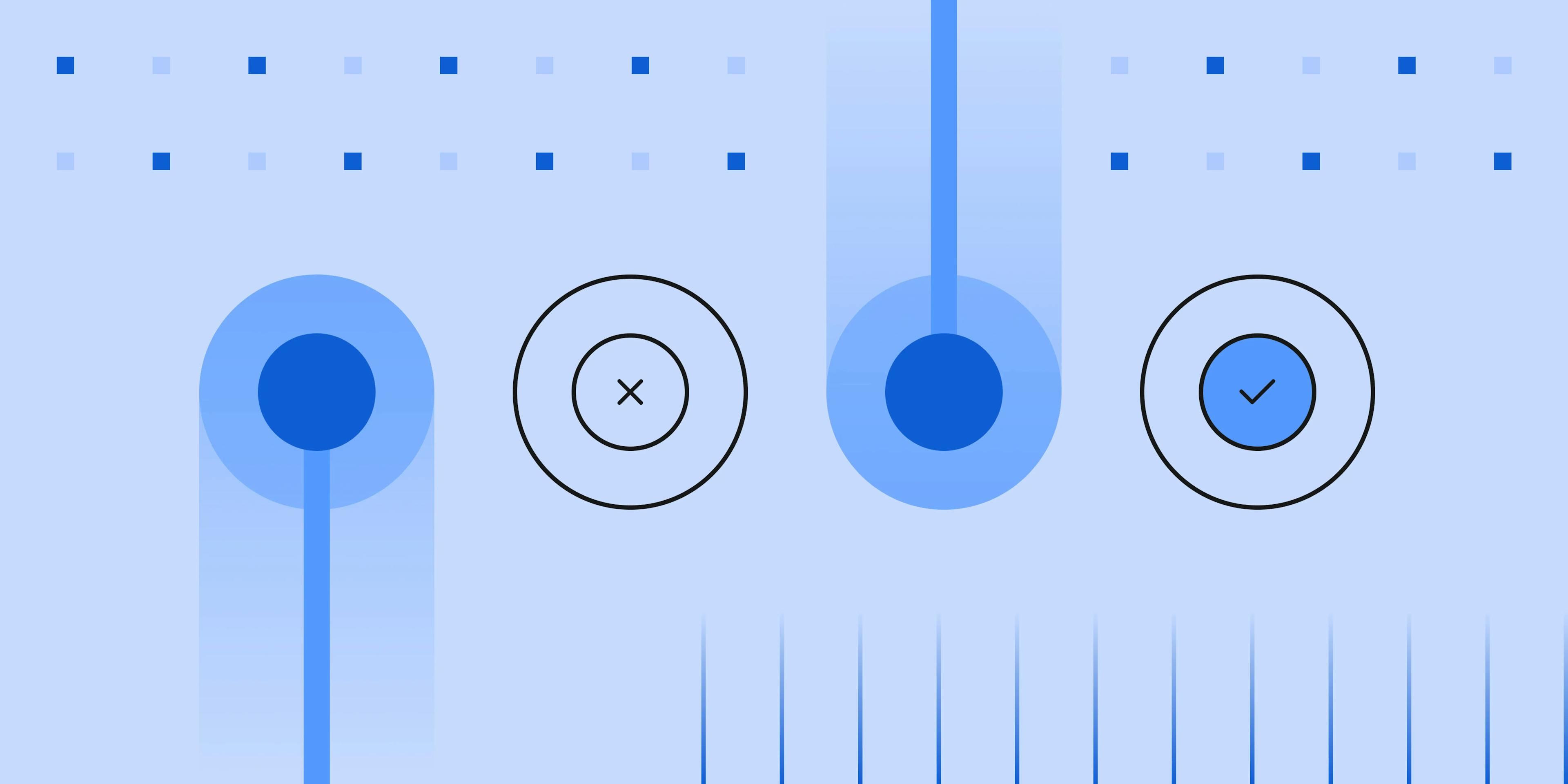
First Party Cookies - A Good Evolution
By Brooks Patterson, Tom Shelley
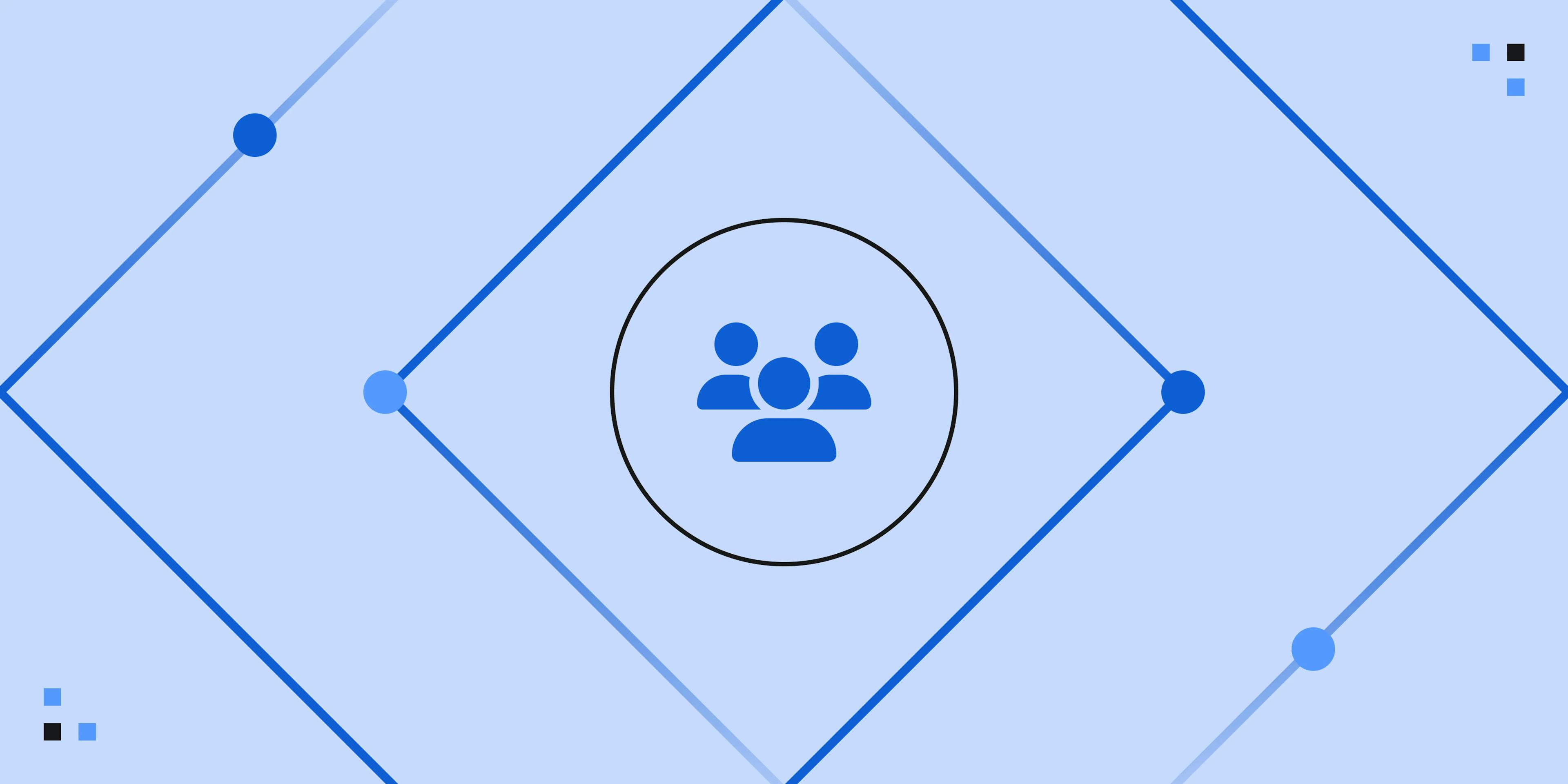
Why data teams must separate support work from development work
By Matt Kelliher-Gibson
Get the newsletter
Subscribe to get our latest insights and product updates delivered to your inbox once a month