Feeling stuck with Segment? Say 👋 to RudderStack.
Blogs
How RudderStack Profiles Accelerates Time to Value for AI/ML Models
Written by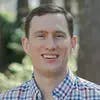
Brooks Patterson
Product Marketing Manager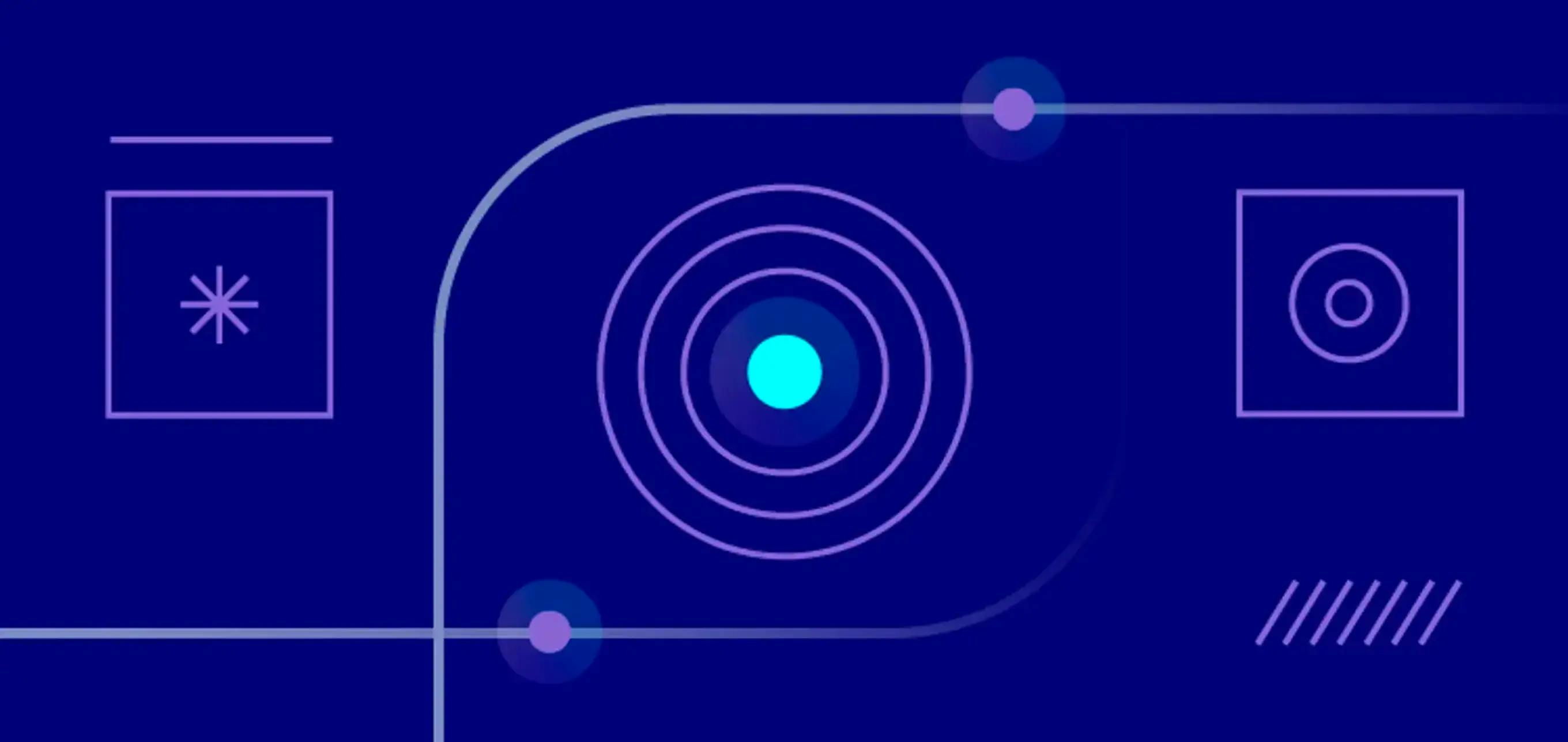
Data science teams have the potential to drive competitive advantage like no other team. In today’s hyper-competitive market, it’s becoming harder to differentiate. The only strategic advantage left for many companies is their ability to build hard-to-copy algorithmic capabilities fueled by proprietary assets – specifically customer data.
These AI and machine-learning projects have the potential to set your company apart and deliver transformative results. Mobile gaming company Wynn Slots increased player revenues by 25% with a retention marketing campaign fueled by their churn prediction model. However, shipping AI/ML capabilities is not easy. It involves difficult work that spans the data stack and involves multiple teams, even if you start with a pre-built LLM.
Getting to the good stuff that drives results requires a strong foundation. Before data scientists can work their magic, you must do the hard data engineering work of collection, unification, and making data available to model. For the average company, this work is inefficient at best. For many companies, it’s a total blocker.
But delivering ML capabilities doesn’t have to be out of reach or wildly inefficient. Keep reading if you’re a data leader, data engineer, or data scientist tired of the tedious, repetitive work required to ship ML capabilities. In this piece, we’ll explore how RudderStack makes rapid experimentation possible for every data science team.
Better data, better models
AI and ML capabilities can deliver astounding results, but much foundational work must be done before data is ready for actual Machine Learning. Time to value for these initiatives can be long, and time is a luxury most teams can’t afford. Especially since machine learning capabilities are most effective when implemented with an iterative, experimentation-based approach.
What makes time to value such a challenge? ML models are only as good as the data they’re fueled with. Models built around and fueled by incomplete or stale data don’t create value and can erode stakeholder trust in the data function.
On the other hand, models running on clean, up-to-date data encompassing the whole customer journey are powerful value creators. Deliver these, and your stakeholders will be singing your praises.
However, one does not simply deliver clean, comprehensive, unified data. First, you must overcome the challenges of data collection and unification.
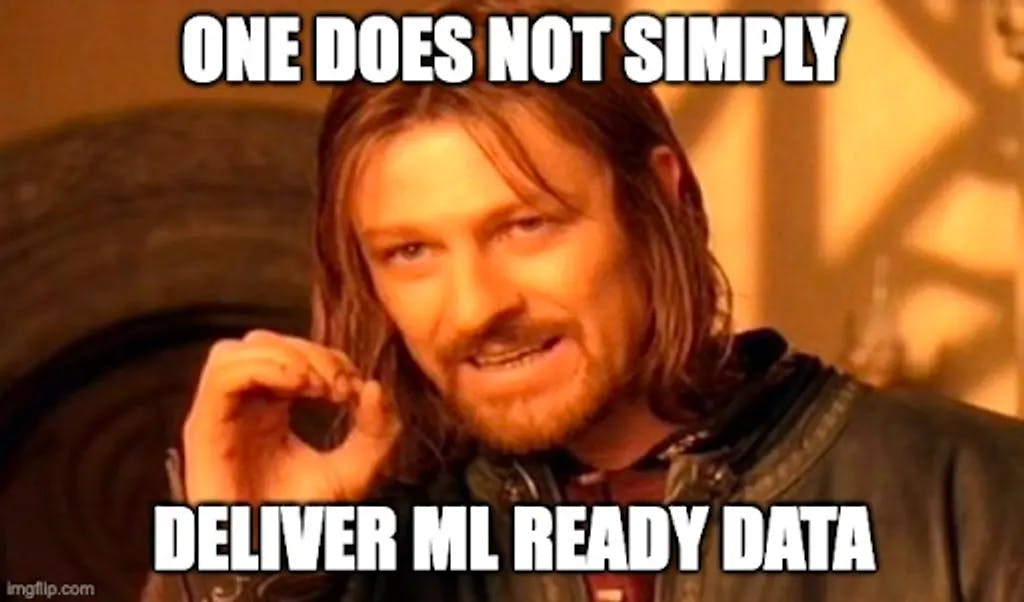
Driving results with ML capabilities starts with data collection from every relevant source. This typically involves getting data from a complex, fragmented ecosystem and centralizing it in a data warehouse or data lake. With multiple web properties, mobile apps, and SaaS tools collecting or generating valuable customer data, even this first step is a formidable challenge for most companies.
We have an eCommerce website, Shopify platform, iOS and Android mobile apps, a subscription service platform, and we have tens of millions of IoT devices at the same time.
—Wei Zhou, Director of Data Engineering at WyzeHowever, getting all the data in one place isn’t enough to power transformative ML capabilities. ML with customer data is all about getting to know your customers better, so once it’s collected and centralized, you need to unify it into complete customer profiles.
Unification involves identifying anonymous and known users under a canonical identifier, creating an identity graph, and finally computing user features on top. This work typically requires writing and maintaining excessive amounts of intricate SQL, and it’s where many teams get stuck. In the worst cases, data science teams end up replicating this data prep work in Python, meaning the work is done twice.
We spent all our time matching customer behaviors to their identities. Our engineers had access to different event logs but couldn’t tie everything together or discern the nuances in user actions to trigger personalized recommendations on all our platforms.
—Wei Zhou, Director of Data Engineering at WyzeThe laborious work involved in solving data collection and unification makes it difficult to deliver acceptable time to value for ML projects. Further, if you solve these challenges haphazardly, your ML capabilities will produce lackluster results.
With RudderStack, there’s an elegant, trustworthy, and time-efficient solution that solves challenges across the data lifecycle that feeds ML projects.
A better way to deliver better data
With our Warehouse Native Customer Data Platform, you can automate data collection and unification dirty work to create a strong foundation for any kind of ML use case. Plus, you can streamline handoffs between data engineering and data science so everyone can focus more on innovation and less on laborious data cleaning and modeling.
RudderStack handles the tedious user behavior data collection work for you with its Event Stream pipelines. You can use our extensive library of SDKs to collect data from every source – with a standardized schema – and centralize it in your own cloud data warehouse. Our real-time transformations make it easy to apply data quality measures in flight.
Then, once you’ve centralized your data in your data warehouse or data lake, our Profiles product takes that data from the entire customer journey, automatically generates an identity graph, and models it into complete customer profiles. Its declarative approach makes it possible to quickly generate a reliable customer 360 and enables you to develop and update user features without intricate SQL. Our new Predictions product can even handle baseline modeling for churn scores and lead scores right in your warehouse.
When you have the power of RudderStack in hand, you can blast off right away. It’s so much easier to build a machine-learning model once your designs are driven by clean data, useful user features, and 360 customer views.
—Wei Zhou, Director of Data Engineering at WyzeWith comprehensive data in your warehouse, modeled into complete customer profiles, data scientists have a solid foundation to build from. They can quickly access model-ready data to train and deploy new capabilities.
Better models, better results
When you fuel your models with clean, up-to-date, comprehensive data about every customer, your models can make more accurate predictions. More accurate predictions enable you to build differentiating capabilities that can’t be copied because they’re built on top of your proprietary data. Customer data can fuel models for many ML use cases, including:
- Churn prediction
- Recommendation engines
- Fraud detection
- Customer lifetime value
- Attribution
Moreover, when you’re able to move quickly and experiment, you can make frequent optimizations to your ML models and create new ones to solve additional business problems. Your data science function can move from operating on its heels in a service capacity to rapidly experimenting to drive innovation.
Now, RudderStack provides the user profiles and Customer 360. We can deal with the data with less complex queries and automate all the dirty work to focus more on research and updates.
—Pei Guo, Senior Data Scientist at WyzeCustomer story: Accelerating model development at Wyze
The Wyze data team used to struggle with dirty, incomplete data and cumbersome wrangling. Their AI team couldn’t move quickly, and its impact was limited because models weren’t running on clean, comprehensive data. Then they found RudderStack.
The team at Wyze transformed their data practice by implementing RudderStack to solve data collection from a highly complex set of data sources. They’re now collecting standardized events from every source to feed models with data from their entire customer journey. They’re also free from the tedious work of matching events and identities because RudderStack Profiles takes care of that for them.
As a result, the entire data org is moving faster and collaborating more effectively. The data engineering team increased productivity 10x! A seasoned engineer can now define multiple events and automate 50+ new user features in a matter of hours – that used to take weeks for the team to complete. These massive productivity gains extend to the AI team. Because they’re getting better data to start with, and it’s easier to quickly define new features, the AI team’s productivity has tripled. They’re training and deploying models with velocity, enabling rapid experimentation and more effective optimization.
Ultimately, these gains drive business results, pleasing the data org’s stakeholders along the way. Now, the marketing team at Wyze ships 3x as many ML-fueled campaigns as they used to, driving increased conversions.
Get to powerful ML models faster
To enable more powerful ML capabilities, you must find a way to efficiently deliver complete, unified data. If you’re bogged down with wrangling, cleaning, and modeling and feel your ML capabilities are underdeveloped or ineffective because of it, RudderStack can help.
Schedule a demo with our team today to learn more about how RudderStack can do the dirty work for you so you can focus on creatively solving problems with rapid experimentation.
Deliver more powerful ML models, faster
See how you can use the Warehouse Native CDP to overcome data collection and unification challenges to fuel more powerful ML capabilities.
Recent Posts
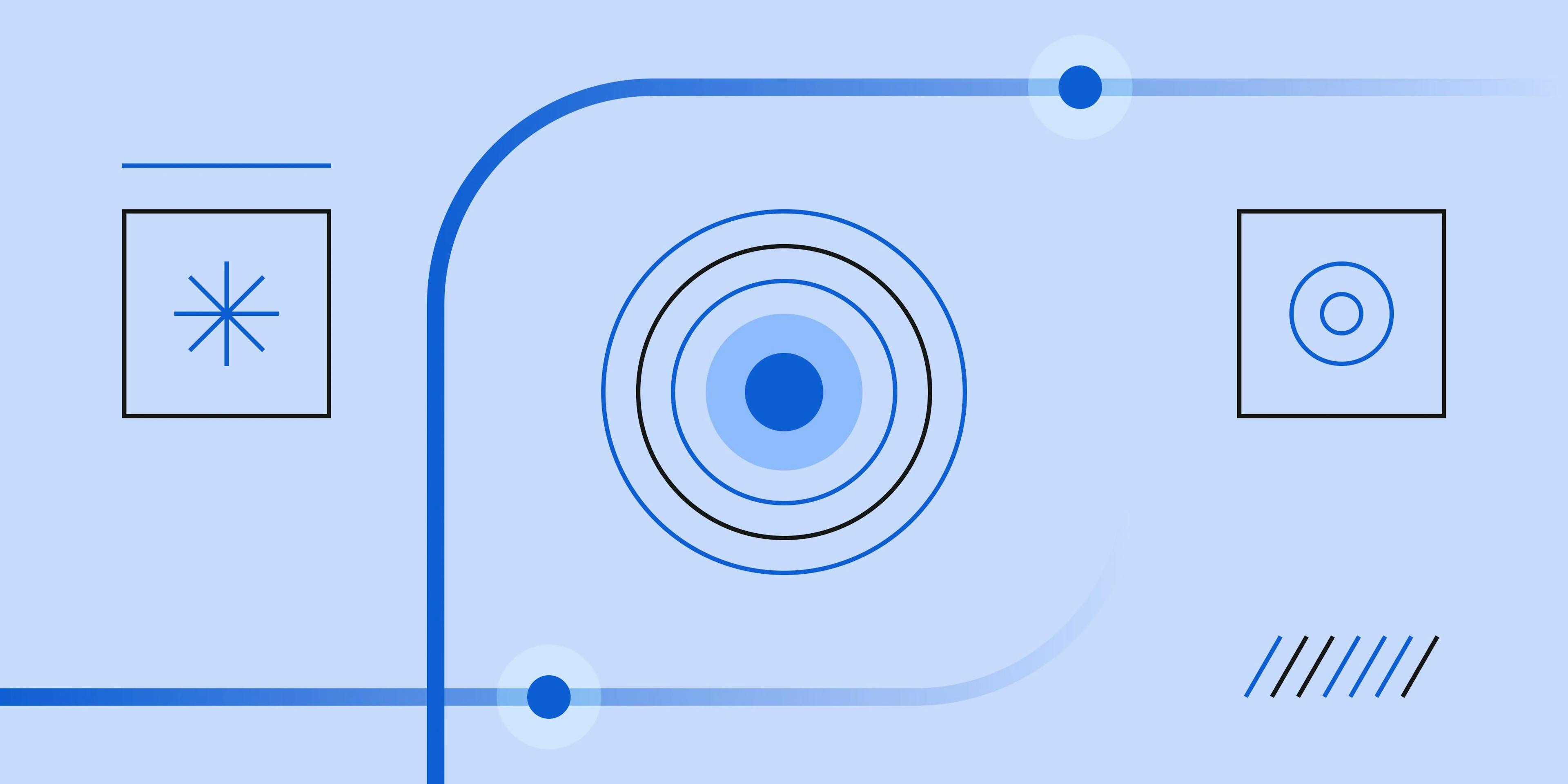
Addressing Segments limitations: from data storage to data strategy
By Mackenzie Hastings
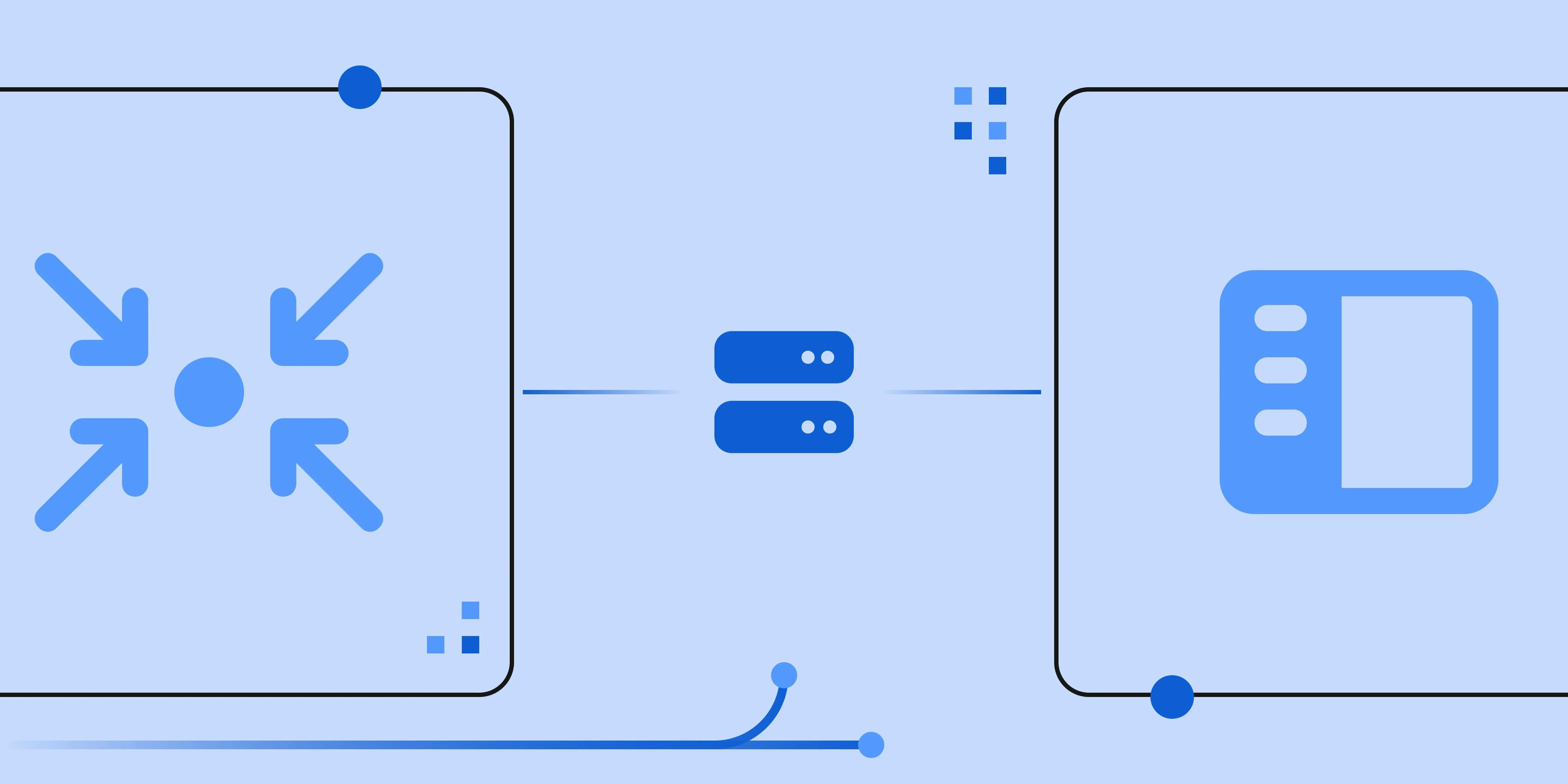
Feature launch: Append and table skipping for warehouse FinOps
By Badri Veeraragavan, John Wessel
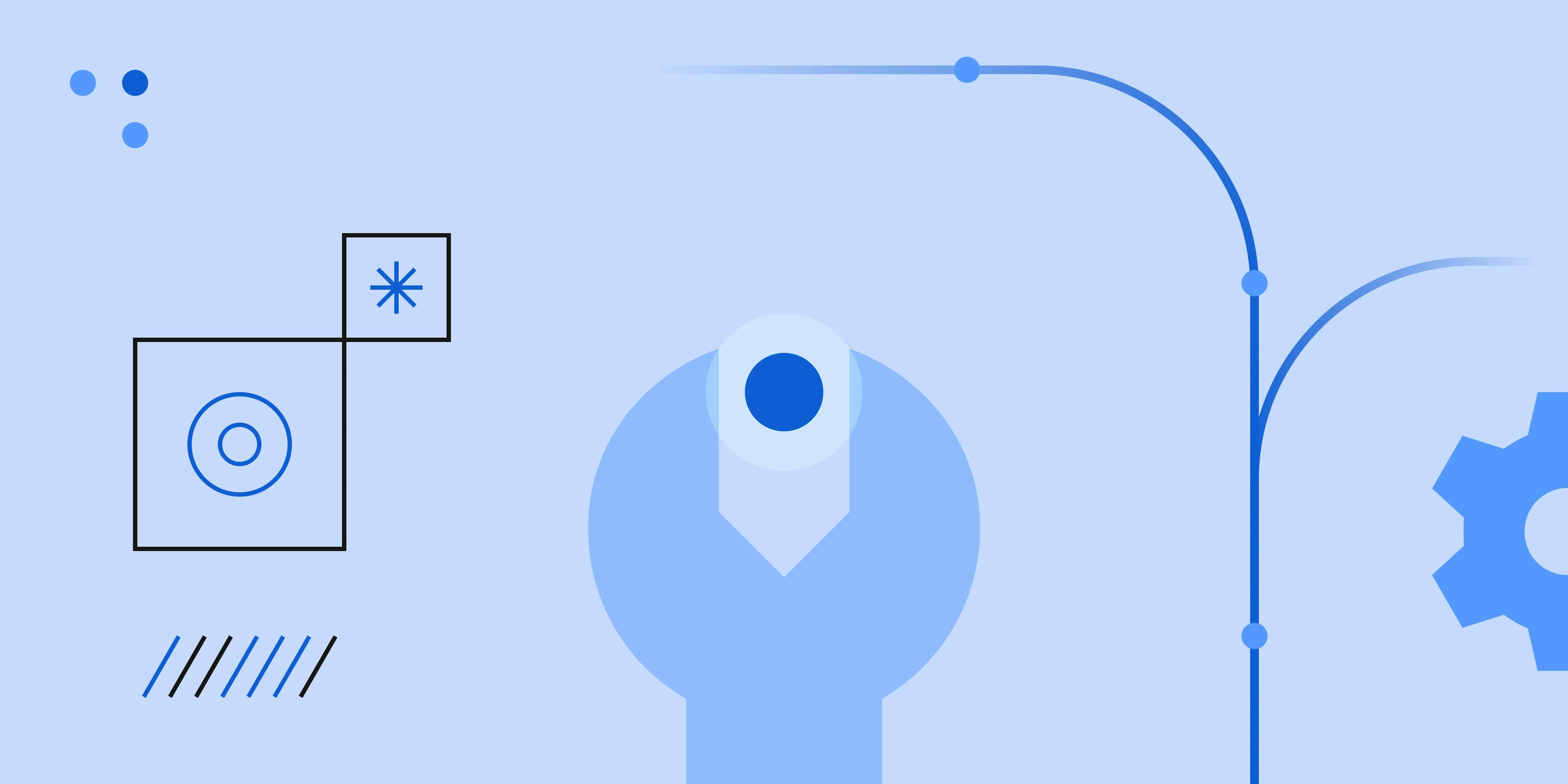
Building the Data Foundation for AI
By Soumyadeb Mitra
Get the newsletter
Subscribe to get our latest insights and product updates delivered to your inbox once a month