Feeling stuck with Segment? Say 👋 to RudderStack.
Events
The Customer Data Stack Journey: Architecting a Scalable Stack
What we will cover:
- A long-term view of the data stack: what are the problems at scale that early stage companies don’t think about?
- The essential toolset: what does every company need, regardless of scale?
- How business models, i.e., B2C vs. B2B, influence data stack architecture and tool choice
- Evaluating near-term and long-term costs
- Beyond the tools: the other critical components of a scalable data stack (data engineering resources, team structure, executive buy-in, etc.)
- Q&A
Speakers
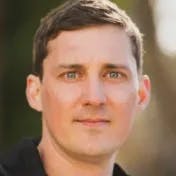
Eric Dodds
Head of Product Marketing
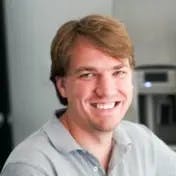
Alex Dovenmuehle
Co-Founder at Big Time Data
Alex is obsessed with driving business impact with data and automation. He's always looking to create automated and scalable business processes, supported by software and data, that allow businesses to scale their operations.Transcript
Eric Dodds (00:00:00)
A couple of minutes past the hour, so go ahead and get started, officially kick this thing off. So I'm Eric Dodds. I lead customer success at RudderStack, which we'll talk a little bit about that tool in the context of your webinar. But today what we're going to talk about is the data stack journey. So we have a really exciting guest, Alex, who you've already heard me bantering with. And we'll dig into his background a little bit, but wanted to give a quick overview. So the data stack journey is really about how in the age of data proliferating inside of an organization, the data that's collected and produced by an organization, one of the big challenges that businesses face is all of the effort, processes, and tooling that goes into having a data stack that works across their company, across teams and helps them build competitive advantage.
Now, the challenge is that businesses change. So what we're going to talk about today is how to architect a scalable stack. So what are the tools that you need to put together at various stages of growing a business, relative to your customer data stack? And what does that look like through the life cycle of the business? So here are the things we'll talk about. So we'll talk about why this stack needs to be dynamic. We'll talk about a long-term view of the stack, so what are the problems that we typically see at scale? We'll talk about the stages of companies as we've broken them out. There are different ways to do that, but we've put together a simple framework to walk through. And then we're going to talk through the toolset and cost at each stage of the business. Briefly touch on business models and how those influence the stack. And then we'll touch on things outside of the stack itself in terms of team structure and other stuff like that.
So without further ado, I would like to introduce Alex. And you have a really interesting background as an engineer in several different contexts, but you are currently a consultant at Big Time Data, which I want to hear about. Before that, you were at Mattermost and before that, you were in Heroku. And I wanted to point that out to the audience because you have a really interesting perspective. Heroku is a huge organization, of course, part of the Salesforce empire and doing things at a massive scale. Mattermost is really a startup. The large, fast-growing startup, but on the other end of the spectrum from Heroku. Now, as the consultant, you see all sorts of different things. So do you just want to give us a little bit of your background in your own words, and then we can dive into the content?
Alex Dovenmuehle (00:02:53)
Perfect. Yeah, so prior to Heroku, I was basically like a full stack developer, front end, back end. I just like to do it all. I joined Heroku about six years ago, and that's where my data engineering journey began. Like you touched on, the nice thing about Heroku was just the scale of everything was bigger, not only because of your part of the Salesforce borg, but also just Heroku itself had tons of data. They're processing just billions and billions. The number is higher than billions. What's over billions, trillions? I don't know. Many billions of requests per month. So it's a pretty big scale there. And basically got into the data engineering stuff there, which at the time was very not good. We were still using bash scripts and our data warehouse was literally a Postgres database. It was a giant Postgres database with tons of memory and stuff, but it was still just Postgres.
So I ended up moving them to more modern data architecture, and I'll touch on that later as well. And basically, about 14 months ago, we moved to Mattermost. And the idea was, we're going to take everything that we learned at Heroku and just replay the playbook at Mattermost, which when we joined Mattermost, they literally had no data infrastructure at all, as many series A, series B companies do. So at Mattermost, we were able to build their whole data stack, analytic stack, go-to-market automation, and all this stuff that we built. And then now we've recently created our big-time data consulting company, because we saw, "Hey, everybody has all these problems. They're all pretty similar." It's like, "I think we can help all these different customers figure it out."
And that's where this idea of the data stack journey started to form in my head because there are differences between talking to companies that are at the seed stage or earlier, versus like a Heroku size, or even a Mattermost size. There are some differences between how these companies are operating, and what their concerns are and how do you build a data stack that allows you to grow from a seed company to a Mattermost size company, to the Heroku-sized company, and beyond that?
Eric Dodds (00:05:37)
Sure. Love it. Well, let's dig in and let's hear about that experience. So we'll just go back and forth here. I think we've actually already touched on this, so I'll intro it and then would love your thoughts on it, based on what you just talked about in terms of the stages of the company. But really the question is, why does your data stack have to be dynamic? And I think the best way is, Alex and I were talking about this in prepping for the webinar is, if you even just think about the last 10 years, you had companies who adopted, at the time, amazing basically on-prem infrastructure to handle all their data stuff.
And then in a relatively short amount of time, you have this massive migration to the cloud, and then you have the first wave of warehouses. And then the current phase is from early warehouse solutions to Snowflake. So, Alex, you want us to give a brief definition of the data stack journey in that context, right? We've seen a lot of stuff happening, we live in a great age as far as tools and all that stuff, but what is the data stack journey in a concise definition?
Alex Dovenmuehle (00:06:57)
Yeah, I like the quote that we have there. I don't want to read it, so I'm not going to, but definitely take that into your mind when you're reading that. But it's, how do I start based on the size of my company, and the number of customers, and the various things that would make you choose different things? Based on those, what tool should I pick? What technologies? How should I even, from an organizational perspective, organize who owns what and where those things live? And then, how do I leverage all this stuff so I'm actually getting data out of it the whole time? And then how does my data stack grow with me so that I'm not having to go back and do all this rework, because I made the wrong decision five years ago, and now I have to spend a year rewriting everything to get to that next to modern data stack?
Eric Dodds (00:07:55)
Sure. Well, let's talk about the problems at scale. So this is something you've seen directly. And why don't we just walk through each one of these? I can give a brief explanation, and then why don't you talk about briefly the way that you've seen that play out at scale when it becomes a real issue. So internal tools become burdensome and costly to manage. We see this a lot where you're evaluating tools and you say, "We have a little bit more of a customer need. We're just going to build this data infrastructure ourselves." What does that look like when it becomes problematic at scale?
Alex Dovenmuehle (00:08:32)
Yeah, so obviously I think there's a few things. A is, can your custom solution actually satisfy all the needs that you actually have for it? Can you actually execute and build that, A? And then B, it's like, "Well now I have to pay these highly paid engineers to go build the thing. That takes time." And then it becomes a technical burden. I won't say debt because it could be amazing, but you know what I mean? Somebody has to know how to operate this thing. Somebody has to be monitoring this thing, make sure that it's working. If the person who built it left or whatever, well, now they have to train, and maybe they don't understand the full context. There are just layers and layers and layers of it that really can bite you.
So I think being able to pull something off the shelf, and I don't want to mention RudderStack already, but I think what's nice about RudderStack is, it's just having the data warehouse as the center of it, it's like, "Okay, we're getting the data into the data warehouse. Now, okay, I can control the data warehouse and I can make everything work in there." So I can still build those custom things that maybe I thought I needed elsewhere, but now it's just like, "Oh, it's in my data warehouse. I'm doing stuff with that all day."
Eric Dodds (00:09:52)
Sure. Yeah, I mean the way that we refer to that a lot is, there's a point at which you're building a product, like a data infrastructure product, and that takes away focus from the product that you're building for the customers who are paying. Lack of unified data, I think this one's pretty simple, and everyone's experienced this. Data gets trapped in different silos.
Alex Dovenmuehle (00:10:16)
Yeah, and I think the worst iteration of this is when you have ... Let's say you have Looker or some data visualization tool, and somebody goes and runs a report that says like, "Oh, this customer paid us X, Y, Z amount of dollars last month." But then somebody else, some sales guy goes into Salesforce and he's running his own reports in Salesforce and it says, "Oh, he only gave us this much." And then now people are like, "Well, which one do I trust now?" And the sales guys can be like, "Well, I like Salesforce, so I'm just going to trust Salesforce." And then the customer support person is looking at a totally different view.
So being able to, especially at Big Time Data, we really think about getting that data into the data warehouse so that you can have those real sources of truth that says, "This is the real source of truth." And then using reverse ETL to get that data to those other systems so that the data is consistent across all those systems. And then that means people are trusting the data, they're going to the right places for the data and all that kind of stuff.
Eric Dodds (00:11:20)
Sure. A single view of the customer, customer 360 are just buzzwords that are thrown around so much, but it really is a big challenge for companies who ... Really it stems from lack of unified data. So you have problems around different versions of the same customer record, essentially.
Alex Dovenmuehle (00:11:44)
Yeah. I think everybody's trying to crack this nut, and I think a lot of people are not that good at it. And that's something that we try to bring to our customers. That's something that we built at Mattermost is, give us the customer 360 that can show you, "Here's all the sales metrics about this customer. Here are all the customer support tickets and stuff like that. Here's the product usage stuff." All in one place where they can go and see that kind of thing. And yeah, as you said, if you have all this stuff siloed around and people are, again, using different views of the data, it just gets very unwieldy and really inefficient I think is the term, because then you have to have people running around trying to be like, "Well, what's the real answer to this question?"
Eric Dodds (00:12:29)
Sure.
Alex Dovenmuehle (00:12:31)
That kind of stuff.
Eric Dodds (00:12:32)
So collection limitations due to cost is an interesting one. Do you want to briefly touch on that? And that's actually something that, that very first time we talked, you brought it up and that was a problem you were solving at Mattermost.
Alex Dovenmuehle (00:12:44)
Yeah, so when I joined Mattermost, they were using Segment at the time and they had ... Because of the cost of Segment, they had to change their code to limit how much product data they were sending through Segment because it was just becoming like tens of thousands of dollars a month or something. And this was a few years ago, so they were like, "We can't be spending this." Because the marginal value of one event really isn't that high, it's really when you get to that aggregate level and where you have enough data to make those ... Especially once you get into the machine learning, and statistical modeling and stuff, you need a lot of data to be able to get the good stuff out of that stuff. And paying so much money just didn't work.
After I basically built the foundation of the data infrastructure for Mattermost, the next thing to tackle was, "Well, what are we going to do to get more of this data? We need this data, and Segment isn't cutting it." So that's where RudderStack came in, and not to toot your guys' horn too much, but it was ... From an engineering perspective, it was a pretty seamless process to get us transitioned over from Segment to RudderStack, which was really nice because I'm kind of the new guy coming in and being like, "Hey, engineers, you need to go do all this stuff." And being able to say, "Hey, here's exactly what you need to do. It's not really that complicated." And they were able to get it done.
And I think we had it done in a couple of months, and honestly, most of that is just because Mattermost, at the time, had a release schedule that was only once a month. So I had to have a big lead time to make sure it got into the release. Now it's like any time a product manager releases a new feature into the product, we're like, "This doesn't go into the release unless it has telemetry associated with it." And we don't have to think about the cost of it, it's just like, "Yeah, you need the data. How are people using this feature that I'm implementing," and all that kind of stuff?
Eric Dodds (00:15:02)
Yeah. Well, let's tackle the last three together because I want to be conscious of time, but processing and transformation aren't abstracted and flexible, which leads to data quality issues. And then analytics ultimately becomes a cleanup team, not actually providing business insights. You just want to get us a brief overview of what does that problem looks like and feels like at scale?
Alex Dovenmuehle (00:15:25)
Yeah. Well, I can tell you the analysts hate it, and that leads to some burnout and people leaving and stuff like that. Yeah, so basically if you can have ... And we'll get into DBT, which I will talk about at length. Just remember the name and we'll get to it later. But being able to have that centralized place and where the analytics engineers and data engineers are the ones that actually do all the logic because they're the ones that are going to know the data across the organization better than anybody. So they're the ones that are going to be able to combine it into actual useful models, that can then be used by the rest of the organization outside of it.
At Heroku, we had times where these marketers, marketing people, which nothing against marketing people, but they're not SQL experts. They don't want to be, they're never going to be. And they're trying to modify these SQL queries to give them their audience for some Facebook ad or whatever it is. A, it doesn't scale. B, it breaks down all the time. And then C, they come to the analyst team and they're like, "Hey, this doesn't seem to be working. Can you help figure this out?" And it's like, "Well, this is dumb, but you should have used one of these things we already had built or whatever." So yeah, that's definitely a big thing.
Eric Dodds (00:16:51)
Sure. All right, well, let's quickly ... So those are some of the problems at scale that you've experienced at Heroku and Mattermost and seen in your consulting. Let's quickly talk about companies’ stages. So I'll just run through these quickly. These are pretty straightforward to people, but you have the first step. So this is the proverbial company in a garage or a couple of founders. They come up with a product and they do everything. They're doing sales, they're probably building the product, and are handling all of the business sides of things as well. And usually, the technology is pretty primitive. On the scale as well from this standpoint, doesn't necessitate a lot of technology. When someone signs up for the product, you probably have Terminal running on your computer and you can see the sign-ups coming in, and you're cheering every time that happens.
As you grow, you go into the seed stage. Usually, you're raising some money around here and building out teams. So the founders are still really involved, but you start to have teams that layer on additional technology. Usually, this is going to be a product team layering on technology for the product, whether that's analytics or other product tools. And then sales and marketing are most [inaudible 00:18:11]. And this is where you start to see additional tools layered in. Usually again, pretty limited, maybe some analytics tools and maybe some marketing and sales, SaaS tools. But again, fairly limited and you're starting to do a little bit more advanced analytics. You have a little bit more data, but a still lighter duty.
Product market fit, that's a whole other conversation for another time. Let's assume that you found product-market fit, or you're in a stage where you're finding it and things seem to be working and you're growing quickly. You have thousands of customers. Of course, this depends on the business model, but you have a huge amount of volume. You're probably a couple of million in revenue. I would describe this stage as you're starting to ask deeper questions about the business, both that are rearward looking, so how did we get here? And that is forward-looking. How do we grow from here? How do we need to change? And that crosses multiple different vectors of the business. So if you're an e-commerce company, you're looking at products, product mix, user experience, all of those sorts of things. If you're a SaaS company, you're looking at product experience, activation. In both cases, you're heavily analyzing the marketing funnel and the customer journey.
And then if you continue to grow, you get to the mature stage. So you're a big player in whatever industry you're operating in, and you're one of the main choices that people are going to. So now you're dealing with pretty significant scale, and here you're dealing with situations where data can move things a little bit, but that makes a huge difference on the bottom line. So we're talking about a 1% increase in the number of activated users, having a massive change in the bottom line of the business. And the reality in today's world is, you need a significant amount of clean data in order to understand how to create those optimizations.
And then Alex's pet name for the last category, which I love is, your grandma's heard of them. And I think we'll just use a quick example here. Netflix is the classic example here. The building literally some of their own toolings internally because they're facing problems of scale that have literally never been faced before because it's a new business model and new technology. Anything I missed there, Alex?
Alex Dovenmuehle (00:20:46)
No, no, no. I think that's totally good. Totally good.
Eric Dodds (00:20:53)
So we just wanted to cover these quickly because, in terms of the data stack journey, it's really important to understand the needs that you have at these different phases because there are challenges with layering on too much complexity too early. If you're seed stage, you don't need to be looking at building your own tooling like Netflix, but at the same time, if you're a mature company, you do need a pretty robust stack in order to meet the needs of the business from a data perspective.
So let's quickly talk through the core data components. I'll run again through these, and I know I'm talking, but then I'm going to hand it over to Alex and you can take us on the journey, which is going to be the best part of the whole thing. Data warehouse, this is the center of the stack, and there are lots of things around the customer data stack that are related and connected to these things, but as Alex and I were preparing for this, we wanted to focus on the core pieces that every company needs, not necessarily the cloud SaaS applications that are commonly conducted. So what are the core pieces of the data stack?
So data warehouse, this really should be the center of the stack. Alex will talk about that. That means that all your data can both be collected in one place, but then accessible to build out the systems and technology and teams downstream. Data transformation, we talked a little bit about this earlier. It's something that becomes a major problem at scale in terms of abstracting it and making it flexible, but this is really the process of business produces and collects all different types of data. And when it hits downstream destinations, where it's used in certain ways, it often needs to be in a different format, or cleansed or changed in some way to be useful as it moves through the system.
Visualization, this is simple, translates data really to be used for humans, most often in analytics context, but all sorts of other ways as well. The event stream piece is getting user behavior data, so that's websites and apps. And of course could be other things like point of sale systems, et cetera, but most commonly websites and apps. ETL/ELT. This term has been a buzzword lately with the reverse component, but we'll call this traditional ETL that pulls tabular data from a cloud app into your warehouse, is what we're referring to there. Reverse ETL, which is the new kid on the block, sending data from your warehouse to apps and other destinations in the stack. Really cool, very excited about that technology.
And then data governance, and this is a broad term, but for the sake of simplicity and time, we're packaging a couple of things in here. One would be keeping your data clean across the entire stack, and then also having an eye towards the security and privacy component. So again, that can mean different things, but we're packaging that all up into one term for the sake of simplicity. So the moment we've all been waiting for.
Alex Dovenmuehle (00:24:04)
Oh, baby.
Eric Dodds (00:24:05)
Alex, take us out of the journey. So we just started a company, you and I, and we're hammering on the product. What is our stack look like?
Alex Dovenmuehle (00:24:16)
Yeah, so one thing to know about me is I'm a huge Postgres fanboy. So we're not using any MongoDB or MySQL, it's all Postgres, but that's just me.
Eric Dodds (00:24:28)
Dually noted, dually notes. Someone's going to comment about it at the end, by the way.
Alex Dovenmuehle (00:24:33)
I know, right? So yeah, you're probably going to have one production database, the Amazon Aurora, or whatever you want to really get. There are all sorts of options out there. And most of them are honestly pretty cheap for what you get. Not bad at all. And like you said, you're going to have the terminal up and you're waiting for those people to sign up, and honestly, the customers that you do get, the most valuable thing you can do is talk to them, like actually have a conversation and be like, "How are you using this product? What do we need to build?" That kind of stuff. You don't really have any problems with scale. You can have your lawyer go drop a contract, and you can do so much of this stuff manually that you really don't need that much tooling. And you can just run some of these analytical queries, either on a read replica of your Postgres database, or if you're feeling lucky, you can run it directly on it if you want to.
If you can get to the point where you're still in that first step, but you're getting enough data into where you really want to have some analytical stuff going, then I would go to DBT Cloud. And I do want to spend some time talking about DBT, just in general, because you can see how it's literally the entire way through, because I really feel like DBT, which stands for the data build tool, is the best choice you can make early on to ... It can help you with even data governance or some of those things we talked about where people are getting different views of the data and that kind of stuff, to really helps you get all that stuff organized and scalable.
Eric Dodds (00:26:26)
Just to pause there for one moment, for people who watch this video, DBT is a very common tool, but could you just give us a high-level overview of what does it do in the stack? We say data transformation. That may be a couple of clicks deeper than an explanation of what it does.
Alex Dovenmuehle (00:26:44)
Yeah, so high-level, and then we'll go down, basically, they say it's like the T in your ELT process. It's all about data transformation. And really what it is, is an abstraction on top of SQL, which is the one really nice thing about it, because it can be very familiar to analysts, data engineers. You don't have to know how to write code to be productive with DBT. And basically, the way that it works is, you can define data sources, like raw data sources coming in from if you're using Stitch, or you're getting data from a third party app or whatever, into your data warehouse. And then you can build models on top of that. And then the real key is, you can then build models on top of that, on top of that. So you have this whole graph of models that's like a dependency graph that says, "Well, this model up here came from these models, and these models and this." So you can see the lineage of how that data came through.
And what's nice about that is, by building things up in a good way, and you can validate like, "Hey, this model is 100% good. The data is super clean, everything's perfect." Now somebody can build on top of that without having to worry about, "Oh, is my source [crosstalk 00:27:57]." Yeah, and they don't even have to know all the complexities of how you got to that one nice model, because it's all abstracted away from them. Now they can just build more and more useful things on top of it. Yeah, and there's a whole lot of other stuff with DBT. We could literally do a whole webinar on DBT I think, and maybe we should.
Eric Dodds (00:26:44)
We should.
Alex Dovenmuehle (00:28:20)
Yeah.
Eric Dodds (00:28:20)
I appreciate it. No, that's great though. I think if I had to summarize the perspective across the journey, that investment early on is something that isn't required in order to have a decent data stack set up at the first step stage, and maybe even the seed stage. But the early investment will pay significant dividends because you've already built a foundation of models for the business and modified those as the business has grown and changed which will make future work a lot easier.
Alex Dovenmuehle (00:28:59)
Right, yeah. Yeah, exactly.
Eric Dodds (00:29:03)
So we're clearing Postgres and watching it live in our terminal. We're building some basic DBT models as we understand the structure of the business. Visualization.
Alex Dovenmuehle (00:29:13)
Yeah, so visualization, again, I think this is where you can, at least especially the first step. I think seed stage, you definitely need something, but something like Metabase where it's not super expensive because you're not going to be able to pay the, I don't know, 40,000 or 50,000 that Looker might be, even though I do like Looker more than Metabase, but that's once you get to that scale. So with Metabase, the way I would approach Metabase too is to build your DBT models, such that most of the queries that are going through Metabase are very basic. You really don't want to be putting complicated logic into a visualization tool like that. And even once you get to the Looker stage, I would try to limit it. I would try to keep as much complexity at the DBT stage because that's going to be the stage where the people who really understand the data the most are going to have control over that tool and how that data gets generated.
So if you're just doing, in your data visualization tool, your queries become a select star from some table, where this, group by that. That's pretty straightforward. You could move that to any different visualization tool; it's going to be really easy. But if all of a sudden you have some complicated joins, and you're doing outer joins and cross joins, and group buys with having clauses and I don't know, all sorts of crazy stuff that you can come up with. Then all of a sudden you start to, "How do I even know that data's good? That could be crappy data. I don't know." So that's where, as you grow, I think me personally, try to keep as much of that complexity in DBT so that it can be vetted and be like, "This is good. Use this."
Eric Dodds (00:31:05)
Sure, and you can avoid the re-work.
Alex Dovenmuehle (00:31:08)
Right, exactly.
Eric Dodds (00:31:08)
I've been in organizations where you start on BigQuery because you have a data studio. So you're just building all of these SQL queries and BigQuery that drive really good reporting, but then you get to the growth stage or the maturity stage and you realize, "Goodness gracious, we may basically have to overall literally everything. We want to migrate warehouses." I'm sure a lot of our listeners have experienced that where it's just a huge amount of work to go back and do.
Alex Dovenmuehle (00:31:41)
Yeah. We went from Postgres to Redshift at Heroku, and I think it basically took us like a year, especially because you think of all the ... It's not only like, "Oh, can I actually get the data over there and get the queries working," and all that kind of stuff. But then it's more on the people and process things again. Now you have to have people who operate this stuff and then hook everything up again to make it all work and make sure it's good and all that kind of stuff. Again, the whole point of this is, how can you build from the first step to a bigger one without killing yourself with a bunch of technical debt?
Eric Dodds (00:32:21)
Sure. Well, let's talk about the next two pieces, and then we can talk about why we don't have SaaS options for the last two. So event stream. So talk with us about the event stream. You're instrumenting telemetry, and your website and app.
Alex Dovenmuehle (00:32:42)
Yeah, exactly. At this stage, you're not going to have a lot of customers. You're probably not going to have a ton of features that you need to be tracking all the different things about, so you're really just going to have fairly basic stuff. And having like a RudderStack free or whatever, just to, "Okay, I can collect that data." Me personally, I've hated Google analytics the more and more that I've used it, but being able to use that on your ... Being able to use that on your website in place of Google Analytics, not only do you have more control over the data and all that kind of stuff, but you're actually able to get ... Almost you want to pay Google analytics a bunch of money to get really fine grain reports.
With RudderStack, you can get the very low level like, "This person viewed this at this time and they clicked this button and all that kind of stuff." And then obviously that then builds your foundation for ... And also that company muscle memory of, when we release a new feature, we do put in a little bit of telemetry. So that just becomes the habit. With Mattermost, we had to do a lot of people work to work with the QA team, work with the engineering teams. "Hey, you guys need to be putting in telemetry for all this stuff. And here's how you should do it and here's the best practices and all that." It's not like it took us a year or anything, but still, that's like, if you can develop that muscle earlier, I think you can scale better and set yourself up.
Eric Dodds (00:34:24)
Totally. Yeah, I think one, I would describe that. We talked with someone who was a single founder building a product, and they had instrumented telemetry in their app. Still very, very early stage. So we were asking them, "Is there a lot of value there because you don't have a ton of data?" And their response was great. They said, "Well, number one, I know that this is going to be valuable in the future, so I'm baking it in from the beginning. But number two, the limited data I do have ..." It goes back to something that you mentioned earlier, Alex, which is, "It really helps me understand which users I should talk to. Oh, that person's trying to do something and I don't have statistical significance on feature adoption because I don't have enough data, but I can see at a very basic level, people are trying to do certain things, and I can notice." You have that gut feeling, just looking at non-statistically significant data around, "I should probably talk to this person and see what they're trying to accomplish."
And then ETL/ELT, talk about that at the ... And these are really similar for the first step and seed stage, so talk about that set up for first step and seed.
Alex Dovenmuehle (00:35:38)
Yeah, so I think for the first step too, it's probably limited on what, I'll say business tools you're going to need, like a HubSpot. You're not going to be using Salesforce. Gosh, what's that one? Amplitude or whatever. There's a limited amount of tools that you're probably going to want to spend money on, because you're going to be spending money on running my servers, and maybe I need to hire somebody or things like that. You're going to be using Google Spreadsheets or something for leads or whatever.
So once you get to the point where you start adding a few of those on, you do want to get that data. And I'll talk of this at the end level, and then work our way back because I think the end goal that big-time data like we're about is, data can get really, really, really valuable if you can really enrich it with everything from everywhere across the organization. So it's like if you can have that customer’s 360-degree view with the sales, the marketing, the customer success, the product usage, just give me the whole picture. And then all of a sudden, I can start making not only more efficient but also the sales guy has more context like, "How is this person using the product," and all this kind of stuff. So having all that data coming into your data warehouse ends up being ... And really not only getting it into your data warehouse, but then also connecting it across all these different systems, that really becomes the trick that really opens up a lot of things.
And I mentioned pipeline wise, which is like an open-source pipeline tool that, uses Singer.io under the covers, which Singer.io is what Stitch data is built on. It's an open-source data format kind of thing. Pipeline-wise, it's like a library. Actually, used it at Mattermost for just a couple of little things, but it's pretty nice. It's open-source. There's not like a company behind it or anything like that, but I think also with ... Again, to build a full circle for a second, first is, with something like RudderStack Cloud Extract, and then we'll get into the RudderStack warehouse actions. It's like if you could have one tool that can get my data in, not only for my product and my website but also my Salesforce, my HubSpot, my Stripe, whatever, get it into the data warehouse. And then also view the extract. I think to me, that's where RudderStack's full vision becomes realized, and that's for any sized thing, and that's what I've been ... So currently it's like, let's make that the real dream come true across all of these things.
Eric Dodds (00:38:43)
The dream. You mentioned at the beginning, your data engineering dream coming true.
Alex Dovenmuehle (00:38:46)
Yeah, exactly.
Eric Dodds (00:38:49)
I think if I had to summarize the first step and seed and let's talk about reverse ETL because there's a lot of commonalities. If we look at the first, or if we look at a lot of these tools, there's a lot of commonalities. And I think we can briefly touch on why all this stuff scales really well. But I think in the first step and seed, if you think about DBT, a visualization tool, event stream, and the ETL piece, especially with DBT, event stream, and ETL, they're not critical, but it really seems like you're building muscle early on that will pay dividends in your velocity on using data. So even thinking about ETL/ELT in the first step stage, that might not be necessary, but man, if you can build a DBT model that combines event stream data and tabular data and have a decent starting point there when you get to the growth and maturity stages, and you've already done some of that work and worked out some of the issues as the business has grown and changed, you can go multiple times faster than starting from zero.
Alex Dovenmuehle (00:39:57)
Yeah, exactly. Yeah and going on to the reverse ELT thing, obviously, when you're first starting out, you can just hand input data, manual data entry, into some of these systems. It's like, okay, fine you're doing two leads a day, or something, into some marketing campaign. And in fact, most of the time you're probably writing custom emails to your customers and being like, "Hey, I really want to talk to you," and stuff like that. So it's like, okay fine.
Eric Dodds (00:40:26)
This is the founder.
Alex Dovenmuehle (00:40:26)
Yeah, exactly, yeah.
Eric Dodds (00:40:26)
For real.
Alex Dovenmuehle (00:40:27)
Yeah, it's just me. And then once you do get to that next stage, that's where it's like, "Okay, I actually need to have some operational excellence around some of the stuff that I'm doing." And that's where the reverse ELT gets into RudderStack's warehouse actions. And this space has been just blowing up recently, so then you've got the Census, [polytonic 00:40:53] high touch. I know there's probably tons more out there that I should probably know about too, but those are just a list. And one interesting one that I do want to talk about, and again, this is on the maturity stage, but you see that Heroku Connect over there?
Eric Dodds (00:41:11)
Yep.
Alex Dovenmuehle (00:41:13)
I actually like Heroku Connect, but it's only for Salesforce, and you do have to use the Heroku Postgres database, which adds some complexity that's not necessarily where you want it to be. But of the tools for interfacing with Salesforce, it's probably the most advanced, but it also costs a ridiculous amount of money, like 75,000 a year, or something, for a basic whatever. You could think about it. I probably wouldn't. I wouldn't even recommend it, even though I like it at this point, because Salesforce is assimilating Heroku into its borg, and that just isn't going to work out too well.
But yeah, so with the reverse ETL, going back to what we were talking about with the before is, being able to get ... Now that you have more data, let's say you're in the seed stage. You have more of this data, you want to be putting people into marketing campaigns, or making leads for the salespeople or something. And if you can have those things automated, even at the seed stage, and especially at the growth stage, you'll need that. Just being able to have things happen faster. It's like, "Oh this customer is starting to engage with the product." We have a DBT transformation that says like, "Hey, this guy should be in this marketing campaign because they did X, Y, Z things in the product." And then that goes and creates a campaign, puts them in a campaign or whatever and they get emails, or maybe it goes to a salesperson or whatever.
Eric Dodds (00:42:43)
Sure [crosstalk 00:42:45] warehouse, which is, it's almost the, if you build those muscles early on and you're collecting this data in the warehouse and you do a good job on building out your DBT models, the reverse ETL allows you to mine the full value out of that data.
Alex Dovenmuehle (00:42:59)
Exactly. Yeah.
Eric Dodds (00:43:01)
So cool. Sorry, go ahead.
Alex Dovenmuehle (00:43:05)
The only other thing I was going to touch on was just, not only doing that kind of stuff, but it also builds that muscle of, the data warehouse is the source of truth for everything. So then people aren't using all those other tools to get the wrong answer. You know how users can do. They can mess any system up.
Eric Dodds (00:43:27)
You're seeming to indicate that companies have experienced problems over customizing Salesforce, but I don't want to put words in your mouth.
Alex Dovenmuehle (00:43:36)
Dude, I don't even want to get into all the crazy things I've seen people do with Salesforce. It is completely bananas because it's just ...
Eric Dodds (00:43:47)
Another webinar, another webinar. Okay.
Alex Dovenmuehle (00:43:50)
We're up to two more after this.
Eric Dodds (00:43:53)
Two more after this, DBT and sales, Frankenstein Salesforce. Okay, I want to be conscious of time here to leave time for Q and A. So let's cover two things. So we've talked about most of this. When is it time to get a warehouse? So you've identified Snowflake and BigQuery. We can pause on that discussion, just because we'll do the third webinar on that. When is it time to actually implement a data warehouse? This is going to vary by company, but seed stage to growth stage. So what are some of the things that are clear indicators, we actually need to go from our Postgres, or production DB, and then also, when do you need Looker? What are the markers where you say, "Okay, I need a warehouse and then I need Looker?"
Alex Dovenmuehle (00:44:38)
Yeah, that's actually good questions. So this is kind of a joke, but I feel like the first time that you break production because you're running some analytics query, might give you a little bit of hint. Like, "Hey, we need to be doing this a little bit differently." It also becomes when you want to start storing a lot of historical snapshots of data and historical data for a long time so that you can see trends and things like that. Because when you're running a production database, you want to get rid of stuff that doesn't matter anymore, as fast as possible, just for query performance and all that kind of stuff. So once you start hitting those limits of having that data there, it's like, "Hey, let's start thinking about a data warehouse."
And I don't want to discount the idea of, you could use a Postgres database as your "data warehouse" if you wanted to, just not your production, like OLTP transactional database. Postgres can get you pretty far that way. And then I think for once you get to the bigger players, Snowflake and BigQuery, that's where you're starting to run into issues where just Postgres can't keep up anymore. It's trying to aggregate too much data. It's not made for it. It's not really made for the analytical workflow, so it's like, "Okay, I really need to do that." Once you get to the Looker stage, and I have a lot of experience with Looker, so that's why I tend to like it. Is with the way that Looker does things with LookML, a YAML type data format that defines your data model, it not only gives you the data governance because you can have your users in different groups and give them different permissions and stuff so that they can only see certain things.
At Mattermost, we have a board-level view that's hidden away from other people and stuff. So you can do interesting things like that, but then also, it gives you that layer of, you're defining the data model. And what people can basically query from the database, they can't just randomly run some crazy query. It's all pre-baked and pre-built.
Eric Dodds (00:46:57)
Sure, got it.
Alex Dovenmuehle (00:46:58)
And then that enables you to have an interface for the users where they're basically just dragging, and dropping, and doing that kind of stuff. Based on the way Looker works, it generates SQL for you, but it keeps them from getting themselves into trouble with doing joints that aren't correct, or whatever because you're defining it all on the backend for them.
Eric Dodds (00:47:27)
Very cool. Alrighty, well I think I'm going to do one takeaway and then we're going to cruise through the rest of the slides so that we can have some Q and A time because we've had too much fun on this journey. But I will say one thing that's pretty amazing is, if you look at this toolset, we live in an age where the tools are robust and scalable enough to handle a pretty wide-ranging journey in terms of company growth. And it's really about, tools are great, and then how do you use them and implement them to build the muscles?
Okay, a quick note on B2C, versus B2B. So I'll just make a couple of comments and then you can make some brief comments. So B2C is generally heavier on tracking non-paid user behavior and doing front-of-the-stack stuff. So if you think about e-commerce, tons of browsing behavior. Product view is trying to analyze that to get to the moment of truth when someone makes a purchase, gaining similarly. Huge amount of interaction data getting to the point where they pay. And of course, there are important things about that experience, but that can influence cost considerations because it can be really expensive to track a high amount of non-paying users in terms of unit economics.
And then B2B is generally heavier on the backend of it, where you're sending a lot of data to SaaS applications that are used by internal teams. So that's sales, marketing, product, data science, et cetera. And there's also a heavy emphasis on enrichment. So there are certainly really interesting use cases for reverse ETL in B2C. B2B is doing mass enrichment at scale in the warehouse and then syndicating that out to just all these other cloud tools and systems that the teams are using. So those, I think are the two things that came to mind when we talked about different business models. Anything you want to add there?
Alex Dovenmuehle (00:49:28)
Not really. Heroku was an interesting business model that I'll just touch on, because it was like, you're selling to the developer first, and then you're trying to get into the enterprise. That was the whole engine that powered Heroku. So we dealt with both in that way. And I think there's a lot of companies that do go that way, where you're first getting the developer persona to use your product, and then you want to get them to that enterprise bigger kind of thing, but yeah. But otherwise, yeah, all there.
Eric Dodds (00:50:06)
Awesome. Okay, really quickly. I'm going to ask you one question on this and then we'll do the fourth webinar. We're just lining them up here.
Alex Dovenmuehle (00:50:20)
Yeah, right.
Eric Dodds (00:50:20)
Thinking about the data stack journey, how would you grow the team? So when do you hire a data engineer? And then how does that data engineer interface with the other teams in the company?
Alex Dovenmuehle (00:50:33)
Yeah, yeah that's a good question. When do you hire the first data engineer? I would personally say hire them earlier than later. I think some people would try to put it off, and I've seen a lot of companies where it's like, "Oh, this guy kind of knows SQL, so we just had him do it." Which honestly, if they pick the right tools, then they could probably get away with it and be okay. I think building that biz ops team that can really be the center of understanding all the data in the company, where it's coming from, and even more importantly, how it's being used, and where it's being used, and understanding of the nuance of how all that stuff works. That's where the value of that stuff really starts to shine because you can have the coolest data lake, data warehouse, whatever, with the prettiest things or whatever, but then you need to have like the operational piece on top of it that's really using all this stuff to make it work.
Is data a first-class citizen in the company? I think it has to be at this point. Your point earlier about, we've reached this point of tooling where it's no longer only the crazy Netflix's of the world, or whoever, who can deal with all this crazy amount of data. You can pump a crap ton of data into Snowflake, or BigQuery, or whatever and you can reasonably query that stuff, provided you set it upright. But I think more people are starting to understand how to deal with all this data, and I think that's part of where RudderStack is trying to help people, at the same time as Big Time Data, we're trying to do the same thing.
Eric Dodds (00:52:19)
Sure. Awesome, yeah I would file that under probably earlier is better than later tenants, and it's an investment that's going to pay dividends because if you start earlier, the team doesn't have to be massive because they're not doing clean up work.
Alex Dovenmuehle (00:52:38)
Exactly. And honestly, that's a huge thing. We've even seen companies where they have big data engineering organizations. It's like, "Well if you didn't make these bad decisions earlier, you would only need like two or something and it would be fine."
Eric Dodds (00:52:55)
Sure, sure. Alrighty, well we have five minutes for Q and A here. Sorry, we crammed through the last couple of slides there, but more webinars to come. So please type your questions into the Q and A field, or into the Q and A box, or raise your hand and I can unmute you. Let me see here. Let me blow up the Q and A box.
Alex Dovenmuehle (00:53:31)
The Q and A box. I've never done release webinars before. It's so much fun.
Eric Dodds (00:53:36)
You like it? You're famous now.
Alex Dovenmuehle (00:53:41)
I know.
Eric Dodds (00:53:42)
Okay, here's one that came in. Oh, we have someone who's raising their hand. Okay, let me ... I'll unmute you in just a moment. Let's answer this first question really quickly. There are lots of new tools coming out, how do you know if you adopt something if it's going to be around? This is a really interesting question because there's something coming up on the product hunt, and how do you know you're making the right investment?
Alex Dovenmuehle (00:54:11)
I feel like that's something, like Big Time Data as a company is doing. We're trying to vet all these different technologies and things so that we get into these client companies, we can say, "Hey, we've actually had experience with this. And we see what the thing is." But I think another thing you can think about is ... Well again, and I'm going to preach the gospel of DBT again is, with DBT, it's open-source, so they're not going to shut down shop and you can't use it anymore. So again, that's that thing of, okay, maybe your reverse ETL tool goes away or whatever, but as long as you have all that data modeled in DBT, there are 1,000 other reverse ETL tools to go used that you could migrate through. Really all you're paying for with the reverse ETL tool is, how do I get it from my warehouse to this thing? It's like API interactions. So by having the data clean upfront, just solves so many issues. A lot of stuff. So yeah, that's what I would say.
Eric Dodds (00:55:18)
Love it. All right, I am going to unmute. Pascal, I think this is how you pronounce your name. We'd love to hear your question.
Paschel (00:55:28)
Okay, it's Paschel, not Pascal.
Eric Dodds (00:55:32)
Paschel.
Paschel (00:55:33)
Yeah. So my name is Paschel. I'm primarily a software engineer from Lagos, Nigeria. So I work in a FinTech company, an enterprise-level, would describe it as that. So when I got in. I've had a bit of a background in data science, and then when I got in, so there was this significant amount of data. A lot of it wasn't, aside from ... Since it is a heavy, heavy IT community. We have developers to do it, just they would put together dashboards to see through like transactions and stuff. So apart from all that, there wasn't a lot of analytics going on. And then I had to push for that slowly. So I'm trying to understand here, one of the things I'm still trying to ... I'm trying to get the community and get to understand one, the impetus of this engineering, and then the importance of building a data culture. I'm trying to understand, particularly around where it's like ... So as you've mentioned, where they would fit into helping me at this stage.
So some of the things that I have used lately are, for example, I deal with something to monitor. So we have on-premises servers. And then I used their flow to do like an ETL system that pulls server data daily. So using like Linux current jobs. And [inaudible 00:57:31]. I pull server data, then with an ETL pipeline, I move that data into a base, and then I have a dashboard that it helps keep people to be able to understand what they're doing, what's going on in the servers, and how they're using servers and what changes need to be made. And then I was able to also spin up something later. We are looking at people, how by using people who are users, and how they're transacting over time. So trying to use that to monitor things, to look at things like churn rates and stuff.
So that's some of the things I've been able to ... Some of the low-hanging fruits I've been working on lately. Well, one of the issues I'm struggling with lately is, figuring out the right tools at this stage and the things to work with. Actually, because currently, I'm the ambulatory ... I'm not [inaudible 00:58:25], but I'm [crosstalk 00:58:27]. So I'm trying to be sure that whatever foundation I'm building on is solid enough that if anyone comes in, either when I'm still around or if I'm gone, it doesn't just fall apart.
Alex Dovenmuehle (00:58:43)
Yeah, yeah. And that's actually an interesting case. I think that probably happens more than people would admit as far as having a larger company not having the data expertise, even at a later stage. And then it's basically like a skunkworks project that somebody like yourself is working on. Again, I think, preaching the gospel of DBT all the time is, as hard as the foundational tool and being able to model that data, I think that's where you can really have some quick wins there. I think the other thing I would be thinking about too is, finding the leadership within your company and showing them some of the things that you've built, and being like, "Hey, I built this thing with Airflow, I've got DBT, I'm doing some ETL stuff." And showing them the value of what you're building.
Instead of it being like a side project within your own job, you can be like, "Hey, I think we should really prioritize this. And we really need to, as an organization, get better about this stuff." So that's not necessarily the technical answer to all your problems, but I think using some of this technology, as the basic foundation, and I think using Airflow is a good call too. We didn't really touch on it, but I love Airflow. But having DBT on top of that and being able to show them, "Here's all this value that I can extract out of this data, just by this side project that I'm doing as part of my job." To me, I think that's the way I would approach it.
Eric Dodds (01:00:31)
Great. We're going to wrap it up here. Thank you, Paschel for that. Sorry, Paschel for that question. And we're going to wrap it up since we're over the hour, but very quickly, two things. Number one, Alex, how can someone get in touch with you at Big Time Data if they have questions or a potential project or they want to evaluate a tool?
Alex Dovenmuehle (01:00:57)
Yeah, you can email me at, it's alex@bigtimedata.io, and you can also just go to bigtimedata.io.
Eric Dodds (01:01:06)
Cool. And of course, if you're interested in building pipelines out, you can visit rudderstack.com, sign up for a free trial, or send an email to me, Eric, at rudderstack.com If you have any specific questions. We've kept you long enough, but we have deep a bench of additional webinars in the wings now that we'll be bringing you in the coming weeks. So Alex, thank you for your time. Everyone, thank you for joining, and please email us questions if you have them, and we'll catch you on the next one.
Alex Dovenmuehle (01:01:40)
Cool. Thanks, y'all.