How Data Engineers Work With Other Teams
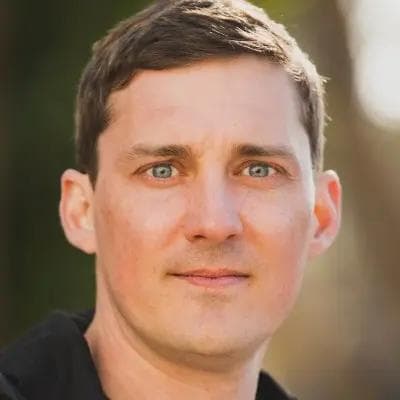
Eric Dodds
Senior Director of Product Strategy
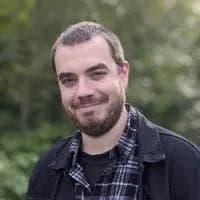
Max Werner
Owner at Obsessive Analytics Consulting
In this webinar, Eric talks with Max Werner, senior data engineer, and owner of Obsessive Analytics Consulting, about how data engineers drive business outcomes through effective collaboration with downstream teams. The session will give you role defining clarity to enhance your day-to-day workflow, and deliver insights into data team optimization.
Max will pull from his experience at companies like Proposify and Warner Media to give you a framework for successful cross-functional collaboration at every stage. He'll detail how the role of a data engineer morphs as a company scales - duties look different, the level of specialization required changes, and the number of teams the data engineer works with changes. He'll introduce helpful data engineer archetypes, and he'll cover the pros and cons of different data team structures.
- The role of the data engineer at each stage: startup, SMB, & enterprise
- Data engineer archetypes: the wrangler, the plumber, & the planner
- Working with external partners
- Pros and cons of common data team structures
- Q&A
Eric Dodds (00:00)
Okay, let's get going. Welcome everyone. We've still got a couple of people trickling in, but we'll go ahead and get started since we're five minutes past the hour. I'm Eric Dodds. I lead customer success at RudderStack and we can tell you a little bit more about RudderStack later, but really what we want to do is talk to Max Werner who has been a friend of RudderStack for a long time and he has been a data engineer in multiple contexts. And the thing we're going to talk about today is how data engineers work with other teams and there are all sorts of different structures that we see as far as data engineers and the way they interact and even the term data engineer itself can mean different things depending the size of the company, depending on the stack and depending on sort of the data needs. So we're going to ask Max all about that and I think we have an intro slide, so I'm probably jumping ahead. Do we need to [crosstalk 00:00:58]-
Max Werner (00:59)
Oh, we do. We do. I mean, you covered it basically all there. Data engineering is like a catch all term for you do things with data, which is like if it's car mechanic that you do things with metal. Lots of things in there.
Eric Dodds (01:20)
Yes. Yes, indeed. Well, let's go ahead and dive in. You want to click to the next slide and we can do some intros since we've already covered that? I'll just kind of give you some accolades, but I'll just give a little bit of background around why we love talking with you and why I'm excited to chat with you about this subject. You've done data engineering in a bunch of different contexts. So for Proposify as a start-up, in the BDB space and you're currently doing some work with Warner Brothers, which is a massive company with massive, massive amounts of data in hundreds or thousands of data pipelines, and then you also do some consulting through Obsessive Analytics and you get to see all sorts of companies. So you've sort of lived and get to experience through consulting just a wide spectrum of different sort of ways that data engineering happens inside of companies.
Max Werner (02:15)
Yeah, absolutely. It gets crazy at times and we'll go into the difference on the start-ups versus the enterprise space, and there's also of course different maturity stages that companies have with data, and there's also of course a number of companies that I do consulting with that kind of they don't have a data stack, they're kind of just on the level of we're collecting some data with Google Analytics and that's kind of it and what do you mean you can do things with data that go beyond running a Google Analytics report? But there's a lot of variety like you said, data engineering is almost never the same from company to company or even within the same company. Oops. I double clicked there.
Right, so different roles for data engineering. I just put SMBs here, I think it's small, medium businesses but that covers start-ups as well. And to start off with a little thing that came across my meme list that kind of summarizes this and it says, dear recruiters, if you're looking for a Java, Python, PHP, React, Angular, PostgreS, Redis, Mongo, AWS and so on, and so on, that's not a full stack developer, that's an entire IT department. And if you look at job postings for data engineers or even data analysts, this is basically the same. You can replace a couple of things here, I mean, instead of Java and Python, it will probably say Python, but instead of Java, PHP it might say Salesforce and those kinds of things. But generally the breadth of the skillset that companies are generally looking for when they're hiring a data engineer is crazy.
Eric Dodds (04:06)
Right, I mean, it's kind of like, okay, you have expertise in SQL, and you can do the odd stuff to connect to downstream tools like Marketo and Salesforce, and you can deploy pipelines on Kubernetes clusters.
Max Werner (04:21)
Yeah, basically you can do it all from start to finish.
Eric Dodds (04:28)
Okay, we know that's not actually reality and sort of the keyword stuffing for engineering focused job descriptions is horrendous and I think that's a whole other webinar topic. And so let's talk through the differences depending on stage and what skillsets do you actually need at sort of the stage of the business.
Max Werner (04:51)
Absolutely. Right, so if we go kind of from left to right from basically small to ever large it comes kind of down to these three different categories, how specialized are you, what do your day to day duties include in the given job and what are the teams that you work with? And of course at a start-up space when there just isn't that many people you are generally not specializing. You actually do mirror that screenshot from before where you kind of have to know a little bit about everything because there is just not a dedicated data operations person that can help you with the Airflow DAGs or Kafka consumers. It's just you kind of have to figure it out as you go, which [crosstalk 00:05:39]-
Eric Dodds (05:39)
And I think in a lot of those situations at least what we've seen is someone who is a leader on the engineering team is sort of responsible for the data stack, whether that's just because they're there and they know it needs to be done or if that's intentional. It's just sort of shepherded by an early leader in the engineering department whether or not they're formally a data engineer those responsibilities roll off to them generally.
Max Werner (06:08)
Yeah, for sure. Of course, that's exactly it, it depends. In some start-ups you have that engineer that is slightly data leaning, or maybe it's even the CPO that kind of does it in his spare time, or you come in and you have a little bit of that server background and you can do it as the engineer, it varies widely. So your duties they are everything or some of those things depending on how small the start-up is. And naturally that means the teams that you work with is all of them, you've got to talk to the engineers to help you with the stack and more so the Devops side of things there you have to talk to the engineers [inaudible 00:06:51] analytics code implemented usually inside the application.
I've seen very few instances where data engineers actually touch the main product codes or be it a web app, a mobile app, a website, whatever. The data engineers often don't interact with that code base I think, usually because they're not trusted but it's a different kind of work, but it helps to have that skillset a little bit, kind of if you know enough about let's say you're start-up has a mobile app and you know enough about how mobile apps work, lifecycle stages for entering iOS apps and these kinds of things that you can communicate more directly with the engineers that actually built the app, that's great.
As we kind of mature a little bit and we get into the small, medium business stage you will naturally specialize more and also of course that means the job postings for data engineers will be more specialized. So if there actually starts to be a data team you're not just the Jack of all trades, you have someone who is more focused on the pipelines, someone maybe that's more focused on maybe even some analysis, or someone that's even directly just specialized on you make sure that Salesforce works from the data perspective, which means [crosstalk 00:08:19]-
Eric Dodds (08:19)
Here's a question for you on the SMBs. So I think the start-up is fairly straight forward in terms of you generally have someone from engineering who's kind of doing a little bit of everything. On the other end we'll talk about enterprise, that's a little bit more straight forward just because the roles become more defined. But in this SMB space there tends to be... And I'm interested on your perspective on this because I know you have done a lot of work sort of in the marketing ops type space as a data engineer. And one thing that we see that's kind of interesting, marketing tends to be the tip of the spear when it comes to data, which makes sense. I mean, they need a lot of data to optimize what they're doing, to understand what's going on, the top of the funnel tends to produce a lot of data, you're driving a ton of traffic and then downstream data sort of subsets of that by conversion rate.
And so in the SMB space, what do you see around sort of there's roles that can blend in different directions? So if someone from engineering has been leading the charge they generally sort of move into a more formal data engineering role that is working closely with marketing, but it's also the case when someone from marketing ops who doesn't really have an engineering skillset kind of glades into the data engineer role and becomes increasingly technical. Do you see any patterns around that? Is there sort of benefits or drawbacks to either approach? I mean, it's not something that you can control, but it's something that we see pretty often.
Max Werner (09:58)
Yeah, for sure. I mean, I've seen it more often on the marketer that becomes more technical side of things rather than the engineer that slides more into the data side of things, just because most start-ups have a hard enough time to get the qualified engineers already so they want to keep them focused on building the main product. And so it's more often in my experience case that the marketers just lots of times out of necessity become more technical.
Eric Dodds (10:30)
Sure.
Max Werner (10:30)
And it almost never backfires because a marketer already has that goal oriented mindset and usually with these data types of things having a goal and working your way backwards of how you get to that goal works out a lot better than just throwing stuff at the wall and seeing what sticks.
Eric Dodds (10:55)
Sure. Super interesting and I think we'll just continue to see I think the blending of data engineering and sort of fill in the blank ops, whether its sales ops or marketing ops depending on the business because the ops roles are just having to become more technical, as apposed to being really good at configuring a single SaaS tool, if that makes sense.
Max Werner (11:20)
Yes, for sure. I mean, as we try to scale more and do more things with fewer people or be more efficient at some point you need to be able to talk to the marketer in terms of the Saleforce objects and fields, rather than saying this your workflow that you put together. So you sort of need to be able to talk with them at a more technical level to really understand what their requirements are.
Eric Dodds (11:50)
Sure. One other thing I would say in the SMB space and then I want to move to talk about enterprise and there's lots more stuff, but just I think a lot of companies find themselves in this space and this is where the lines are blurred the most is leveraging a data warehouse to do a lot of data engineering type work. So in the start-up world you may not be and really in many cases it doesn't necessarily make sense early on to be really warehouse heavy in terms of moving data around, A, because you don't have that much data and B, because a lot of times you'll maybe just replicate your production database from the app and that has basically all of your data points and you can sort of use that as a common thing we've seen with early stage companies.
You don't need to provision an entirely separate warehouse for analytics, you can sort of if you're running PostgreS you can replicate PostgreS and sort of get the data where you need to go and probably doing a lot of just initial manual querying to figure out what's going on. But as you get into SMB space you start to adopt the warehouse, analytics needs grow, you're adding additional pipelines so you sort of get into [inaudible 00:13:07] space and you're starting to pull data in from SaaS applications and join it. But I think when you get into managing those types of pipelines you have to have some level of engineering skillset in order to make everything work because it just crosses so many technical components in the stack.
Max Werner (13:28)
Yeah, for sure. Like you said, [inaudible 00:13:33] move more to the having a separate database, data warehouse depending on the size for the data people just because production transactional databases tend to only reflect the current state of the user or a thing inside the product because that's what the app needs in order to serve the user what they're looking for. It's like if you work on a document inside an app or something you only need that current state. Whereas the data people get more interested into that events stream data that is, how did you get there? What actions did you take? And you can't query that from that production database anymore.
Eric Dodds (14:22)
Absolutely. All right, I am done with my rant on SMBs, let's talk about enterprise and then move on.
Max Werner (14:28)
Yeah, sure. Enterprise, I mean, obviously an enterprise with that I mean when you have a company that has thousands of employees and a large amount of dedicated and very specialized people. I mean, for example, here on the Warner side of things the analytics that I work with has at this point over 100 people whose focus is to varying degrees of specialization data. So that's not the people that made the games, or the movies, or whatever, that's literally just 100 data nerds bunched together.
Eric Dodds (15:05)
That is larger than a lot of companies.
Max Werner (15:08)
Yes! Yes, it is. So of course at that point your specialization becomes very, very focused. You don't need to have an idea of how [inaudible 00:15:17] work, and data modeling works, and data pipelines work because there is dedicated teams for that or where actually they will have a dedicated CRM team that is focused only on it. So you naturally become very specialized and that also [inaudible 00:15:34] reflecting then on job postings and that kind of stuff where you don't have that laundry list anymore but rather we're looking for someone to help out in this specific area, which is why the duties I have here and you go pick one.
And you still need to have an idea of what the other workspaces include just because it helps you talk with these teams on their level. If you have no idea what Airflow does and you have to talk to specific pipeline people that all they can talk about is Airflow DAGs, that makes your job more difficult. You don't need to be able to run that or maintain it by any stretch, but just having an idea of what it is and how it roughly works will help a lot. And actually you only work in your own team most often where there's specific points of contact or stakeholders that might get pulled in, but for the most part if you joined the pipeline team you are working with other people on pipelines and not much else.
Eric Dodds (16:38)
Yip, makes sense.
Max Werner (16:41)
So that would cover kind of the size comparison of start-ups to enterprise and how specialized those are, but now we can talk a bit more about what those specializations are or data archetypes, because you said there's lots of people that are just called data engineers but they all do different kinds of things.
Eric Dodds (17:07)
I love the names you came up with this. So for everyone listening, these are great and I think this will resonate with a lot of people.
Max Werner (17:16)
Yeah, I hope so. I mean, there's three for actual engineering. Generally if you go to the start-up space there is a couple of other ones as well, but for actual data engineering types you have these are what I call the Wrangler, Plumber and Planner. Because in the end on the Wranglers side that's the person that's usually the more expert in the analytics tool, or the Salesforce, or the CRM, or what have you that know that tool inside out and is kind of responsible for making sure that whatever data the users of those systems need gets there. If somebody wants to target a campaign on some user data behavior, the Wrangler would be the one creating the warehouse, building the DBT model and synthesizing that data trades for users to get into that system. On the degree of technical specialization this would be more the lowest end because [inaudible 00:18:25] a complication, for the most part this is SQL. You need to understand your warehouse, your window functions and whatnot and get your data out clean.
Eric Dodds (18:35)
Right. You're understanding data models that come with the various sources and probably have a heavy analyst background or in smaller companies even sort of serving as the analyst [crosstalk 00:18:49]-
Max Werner (18:49)
Absolutely.
Eric Dodds (18:50)
... and maybe writing some reports. So really good working knowledge of the data models but in terms of actual sort of configuration of flows probably not getting into that as much.
Max Werner (19:02)
Yeah, because that's more often... So the Wrangler basically takes the clean data, hopefully clean data, that is in your warehouse that you already know, your lower mainstream data or your catalog data if you mirror things like your CRM or ticket system or something into your warehouse to do some analysis, that's standardized schemas and the Wrangler builds on top of that to give your user profile or account profile [inaudible 00:19:30]. Once there's pieces missing in that chain you get to the Plumber because you need to get data into a tool that you don't have an API for or a built in water connector or what have you.
The business requirement doesn't change, if you can't persuade them from using a specific tool because it doesn't have an integration you need to make that happen somehow and that's why I call it plumbing because you really just connect things from left to right and making sure that everything flows as it should because it's much more bad if you don't do it right. So these are the heavy Python and Note users, those are the people that write Airflow DAGs or Lambda functions of what have you. They build those API integrations if they have to.
Eric Dodds (20:27)
One thing that is interesting when you think about the Wrangler and the Plumber, and I'm just thinking from the RudderStack perspective here, and this may sound funny for me to say as obviously an advocate for RudderStack, but I think there's a common misconception in the industry where sort of the data pipeline or CDP ask tools, the marketing promise is just use this and you just need a Wrangler at that point. If you use this tool all you'll have to do is Wrangle because you'll have all this clean data in the warehouse and then you're off to the races. And the reality is what a lot of companies are trying to do in terms of think about driving personalization, or sort of truly building the customer 360, the reality is even for RudderStack customers that involves tooling that's outside of the scope of what our product provides.
I mean, you have Kafka streams running internally, you have data science pipelines that are driving models, I mean, there's all sort of other components that deal directly with the same data and so it is interesting. I mean, we work with a ton of Plumbers because they're trying to interact with a lot of the data that our product provides and so for companies trying to build through those advanced use cases the promise is that it gets a lot easier, but I don't think you'll ever get away from having to have a Plumber on some level.
Max Werner (21:57)
Yeah, for sure. I mean, you mentioned models for instance, so like machinery there is no plug and play where you direct your events stream, be it Rudder, or Kafka, or whatever into a black box machine running tool and out comes money, that just doesn't exist and that won't exist. There has to be that Plumber that helps get that set up, right?
Eric Dodds (22:25)
Right. Well, Bitcoin mining is maybe as close as you can get where you just point some tech at something and money comes out on the other end.
Max Werner (22:35)
Yeah, that's true. And no, Plumber, that won't go away because there is a million and one tools even under the sun and you from the Rudder side of things can't build a connector, be it a destination connector, for every SaaS tool out there. You can't have a cloud extract like a [inaudible 00:23:00] available for every data source that someone could imagine, because that's just not a scalable process because you have one or two customers that might be interested in that and you're not going to spend all the time on that.
Eric Dodds (23:14)
Sure. And even beyond connectors, and we'll move on to the Planner in just a second, but one last point here, even beyond the connectors there are certain use cases where there's just a high level of customization. So one example that comes to mind is one of our RudderStack customers is doing personalized recommendations via Redis and they're using a ton of the data from RudderStack obviously sort of events stream and products views and you can feed all that into sort of a data science model and then their computing traits and pushing them back into Redis and run those action, it's very cool.
But the way that they sort of are implementing Redis is very specific to their company and so Redis is a great tool but that's not an out of the box plug and play type situation for any company because you're sort of delivering the last mile of recommendations fed by a model and that's really specific to how your app is built and all that sort of stuff. And so it doesn't make sense for every part of it to be 100% automated by some sort of SaaS tool where no one every puts their hand on it.
Max Werner (24:22)
I mean, SAP is the greatest example of that where you're never done implementing a SAP tool ever because it is, like you said, always so specific to your company to your use case and that's the Plumber part.
Eric Dodds (24:39)
Yep. All right. Planner. Sorry, I feel like I'm getting stuck on the middle column and then we rush through the last column. Okay. The Planner.
Max Werner (24:49)
The Planner. I mean, [inaudible 00:24:51] to it. So the Planner, that's going to be it's still an engineer at hart because you still have to have the understanding of how these pipelines work or at least you have to have an idea of how could a data pipeline work from tool A to B, so that's why it's more like the project manager for data things. So the common workflow here is you work at a company and their customer success people go, here's this great new customer success tool that we want to use, we need to have it integrated in our tech stack. Now you need the Planner data engineer here.
They're often part of these decision making teams to whether or not to actually buy that tool because if the tool's super shiny and really useful and the end user, in this case customer success, loves to use it, that's great. If you can't get the data into it then you need for those people to actually use it in a scalable fashion. It's a non starter, it's garbage, you can't use it. It might be working super great for other people, it just might not work for you. I mean, I've been in that position quite often where I had to just veto purchases of certain tools because it wouldn't have integrated either partially or at all.
Eric Dodds (26:13)
Sure. Absolutely.
Max Werner (26:15)
It's basically being like, can we do it?
Eric Dodds (26:20)
We kind of call that shepherding the stack. You generally have someone who's kind of shepherding the stack and they have a really good knowledge of all of the ways that things integrate and they are a huge driver of figuring out what's actually going to work.
Max Werner (26:33)
Yeah, because in the end they're the one responsible for keeping it working, so if they can't do that they are not going to be happy.
Eric Dodds (26:41)
Sure. Absolutely.
Max Werner (26:44)
So a little detail about the Wrangler, I know we've talked about it before, the tools that they're using are more tools-tools like CRMs, marketing automation tools, they're the warehouse users. They most often work with those people that really want to activate data, the marketing people, the sales people to serve together the product people that want to either drive personalization or want to have information on, do people engage with this feature that we built? And that's prime Wrangler space because you collect the information and you get it into your CRM, that's their job.
I always call it translating nerd to English because they most often have to take a non technical requirement that is, I want to know how many people use this to did this sequence of things and they have to translate that into, okay, what's SQL query do I have to run against the warehouse, or what funnel do I have to build inside the product analytics tools in order to get them that answer? That's often difficult and it's most often the gift of what they need not what they're asking for because often they're the less typical stakeholders, they often don't know what to ask for. Especially if you work in a more traditional companies.
Eric Dodds (28:10)
The translation actually kind of happens with the Wrangler. It's interesting, so for the downstream teams they sort of give non technical requirements and the Wrangler has to translate those, but then they're oftentimes where the Wrangler says we actually need the Plumber to provide some sort of infrastructure or feed and so they have to translate that way as well. So it is an interesting role because they translate sort of from non technical to data and then from data to pipeline which is kind of interesting.
Max Werner (28:46)
Exactly. So the Wrangler most often works with the plumber there because unless it's already a plug and play thing and it's just you have to pump a SQL traits somewhere, you're going to have to have that technical discussion of what's the best way to get the data from A to B. So they're most often just a purely supportive function and very reactive because they just get told we want to do this and they have to figure out how to do that.
Eric Dodds (29:13)
Yep.
Max Werner (29:15)
All right. So speaking of the Plumber, those are like I said a little more on the technical side, they always have their terminal open and their code editor open, they work in the cloud platforms so like your AWS, your Azure, Google Cloud Platform, Google Cloud... Whatever they calling it these days. So they know how to use those tools to get the job done. Of course naturally that means the teams they work with this other data people or more heavy lifting engineers because let's say you want to capture some information that is currently not even captured, they would also have to talk with the engineers preferably on their level about where in the app modifications would have to be made to allow for that data collection.
And again, depending on the size of the org. or not they might talk to some other teams as well, but mostly it's the fairly heavy technical people. It makes sense, much more technically focused and they often have to have that business analyst skillset built in because they get these abstract goals that they have to translate it into what are the technical requirements, what's this going to cost, is it actually worth doing that, there might be a direct cost in terms of licensing or just time investment for projects, so that comes often into there. The summary for the Plumber is basically they figure out how to get data from A to C when that B middle part is missing.
Eric Dodds (31:01)
I'll continue my trend of blabbering on the middle option, but one thing here I would say that we've observed a lot is that at a large organization the sort of lay Plumbers is really complex because lets just think about a simple example pipeline, okay, so you have maybe user events being generated in a suite of apps probably like a web app and mobile app and then you have pipelines in the middle and then you have warehouses. That sounds simple enough and of course the stack is far more complex than that, but even in that scenario you're dealing with release cycles, on mobile you're dealing with previous versions of apps, so you have users that are running previous versions of apps, so if you have data updates you have sort of multiple sets of data from historical stuff coming in, you have release cycles, then on the warehouse side you have sync schedules and so at large organizations they have to have some understanding of orchestration because you have sync cycles with different warehouses and multiple warehouses. And so it is really pretty complex, pretty challenging job.
Max Werner (32:21)
Yeah, it absolutely is. I mean, it's personally something that I enjoy doing just because you're forced to learn usually new and complex tools. I mean, that's at least something that I enjoy doing.
Eric Dodds (32:37)
Me too.
Max Werner (32:38)
All right. Well, moving onto the Planner, familiar with all, master of non. And like you said, they're more so they have to have this 1000 foot view that is what's our current stack ques that are shepherding the stack because they have to know what's going on, what's growing and make decisions or recommendations that are like, do we integrate this or not, can we, does it make sense, is it a bad idea? So they most often work with data teams and also external teams. So they are the ones that work on proof of concepts with software vendors, because the CMO might say, here's this great marketing tool that I want to use and then you as the Planner have to go in and talk with the sales person, the account manager from those tools to see whether or not that's even a realistic possibility.
So you have to have a lot more people skills in this one, but still with that data of course focus and background and engineer background to really understand what the implications of adding or removing a piece out of that Jenga puzzle that is a data stack. All right. It's fun. You're doing a lot less coding here, but you're doing a lot more planning and anticipating problems, which has a slight downside that you could often be seen as the [inaudible 00:34:20] here because you have to be the voice of reason that is like, this is not going to work just from the data infrastructure perspective and we need to find something else, which is a hard conversation to have when someone is really dead set on using a specific tool, you just have to say, I'm sorry, I can't help you with that.
Eric Dodds (34:41)
Yep, makes sense.
Max Werner (34:43)
So a lot more project management focus here than code execution or SQL modeling.
Eric Dodds (34:51)
Yep. And probably found a lot more in larger organization where you have a bigger data team.
Max Werner (34:57)
Yeah. I mean, and that's also usually once you grow and you have a data team that grows, you're going to have to have someone that is in charge of that data team, like a data ops manager or something along those line. And the best results that you tend to get is if that person is this Planner, someone who has either done the plumbing or the wrangling before and then moved up into that management role because they understand what their team is doing and the challenges that they're facing but they can then also help more of the strategic side [crosstalk 00:35:31] that stack.
Eric Dodds (35:34)
Absolutely. Alrighty. Well, let's talk about team structures because I know we're getting close to time here. I'll try not to interrupt.
Max Werner (35:46)
We'll try go a little faster then. I mean, there's different type of companies, besides the size there's also types of companies. So agencies is our first starting point here. Often data engineering at agencies, and with agencies I mean marketing agencies, so not generally consultancies but rather a marketing agency that builds websites or whatever, mobile apps or whatever for other companies. If you're a data engineer at an agency you live in marketing because unless that agency's insanely huge they don't have their own department, so you're part of the team and you often have to be that Jack of all trades. You often have to do less pipeline tooling here because at an agency the workflows tend to be more not repetitive, but I guess a little on the repetitive side because you build apps, so you're thinking of what you have to measure and what you have to get out tends to be the same from project to project or at least some more.
Eric Dodds (35:46)
Sure.
Max Werner (36:53)
So I mean, in team structure you often don't have a dedicated data team inside of marketing but you tend to work with other marketers, often the account managers if things go really bad with the clients. If you're a data engineer at a marketing agency and you have to be on a client call that's usually when something went really wrong.
Eric Dodds (37:13)
Yes indeed.
Max Werner (37:16)
Sometimes you work with the developers just if there's things that are beyond your skillset. This tends to also be lots of times the entry level or the entry way into the data engineering world, is to help out with the analytics sides of marketing agencies because since it is a standardized workflows you can learn something and repeat it often and then grow your career from there onwards. Pros and cons of being at an agency. There's a lower barrier entry into this industry, not [inaudible 00:37:54] but the agencies with the data industry. When you have to work with the clients in case of something goes horribly wrong, you learn soft skills because you have to and you can't tell the client, well, this is just a dumb question. It's often frowned upon. And like I said, you have standardized workflows. I put it here as a con because it helps you refine your skillset, if you don't have to constantly figure out a new problem you can learn how to be better at solving the same kind of problems.
The con is that at a marketing agency data tends to not make money, you're a cost center, which means you have an issue vouching for, hey, we need this more complicated tool, or we need to pay for our data stack, we need to have a warehouse, or we need to have ETL tools because since data doesn't directly make money its hard for the agency to pass that cost on and its difficult. And of course the flip side of not specializing as much means you have a harder time really mastering a specific skill because you might be doing a little bit of SQL now, but in JavaScript the next day and so on. So that's agency life. I mean, there's other parts to agency life too but as far as the data engineering side is concerned [crosstalk 00:39:20]-
Eric Dodds (39:20)
Yeah, absolutely.
Max Werner (39:21)
... this is it. Another thing is you can be a data engineer embedded in teams. So this is not an agency but rather a company that makes a product or a series of products. Here it is usually on the smaller side, there isn't a dedicated data team but rather certain teams have a data person on them, especially if you have some sort of sward structure, there tends to be a couple of engineers, a product person and a data person that kind of [crosstalk 00:39:52] off. So obviously where you are depends on which of those ponds you fall into and depending on these ponds it also changes which of those archetypes you are.
Most often if you work with product or support and success you have to either be the Wrangler or the Planner, just because they have very established tools that they work with and usually for established tools there's established pipelines so you don't have to do as much plumbing, it's more of really using the data. On the marketing side you have to be with the Plumber because they want to capture things here and there, or try to activate something in the new different tool, or whatever and you have to just make it work. In the engineering side of course you are the Plumber, sometimes the Planner, but most often the Plumber.
Eric Dodds (40:45)
I think marketing's data demands I think again we kind of said earlier it's the tip of the spear, but just the volume, the complexity, the velocity of testing, it just demands a lot of change, which is good. It allows teams to move faster, but it just requires sort of rewiring things over and over again.
Max Werner (41:06)
Of course and that's the agile mindset that you have to have there. So it's like you have to build something full well knowing that in a month or two whatever you've built is now garbage because either that project is done or the tools isn't being used anymore or whatever, but that's just part of the job there. Right, pros and cons. Obviously if you're directly embedded in the team that you have to work for there's a lot less back and forth because not necessarily right now on account of the state of global things, but you generally sit right next to the people that have the questions for you, so that helps. Which directly translates into you can be more proactive if you don't have to wait until somewhere down the road a problem comes up where somebody else is like, okay, and how are we measuring this or how are we supporting this tool? And you're first question is, what tool, what feature? I've never heard of this.
It tends to not happen if you're directly embedded in the team and of course you are by nature of that more specialized because you're focused on CSM and CRM tooling, you learn the ins and outs of the pitfalls of those tools. On the cons side you could feel like you're on an island because you are not directly contributing to the same kind of output that the rest of the team is doing. If you're on product for instance, the product person works on a feature, the engineers built that feature and you kind of just do data things around that feature. It feels a little islandy at times and it's most often just a modern entry level role. You have a very hard time being entry level going into a data engineering specifically on a CRM team because they're looking for very specialized skillsets for that role.
Eric Dodds (43:10)
And I mean you're kind of jumping a lot of times into a workflow that even though it can be established there's complexity there and so you need to be able to jump in and kind of figure out what's going on and provide value pretty quickly.
Max Werner (43:27)
Yeah, that's usually an expectation of those kinds of roles is that you can provide value very quickly and that you don't take three months to onboard. And of course it requires some non data skills because if you have to work on a specific team that deals with a specific thing, be it the internal product or an external SaaS tool, you need to understand that tool and if that's something that you don't care about then one of those embedded teams might not be for you. Alrighty, as its own team. Well, if you're on your own team, you're on your own team, as simple as that.
It really depends, those team tend to hire it's still always just listed as data engineer, data analyst but you could find out pretty quickly from either the job description or from an interview or just reaching out on LinkedIn what kind of archetype they're looking because its usually very obviously because by the time an org. has its own data team they don't look for a person that can do 30 different data related things at once. They look for, I need another analyst just because we have so much more SQL building to do. And of course if you're in that [inaudible 00:44:44] system as a data team you have to work with everybody else in the org. because the whole point of centralizing that is to have that cluster of data people that then can support everyone else.
Well, the pros, you get to work with other data people, which means you can learn from them and you can bounce ideas off of them, you can talk through roadblocks and hypotheticals with people that deal with the exact same thing that you're dealing with, that is a huge benefit which leads to best learning opportunities. Also, if you're on the more senior side here there's still a great learning opportunity because having to explain your own data stack issues to someone who is newer to that org. helps you really annotate and document some things are second nature to you but seem very strange to a newcomer. And of course you're specialized.
On the cons side, if it's not executed well, and that's usually then on the data ops manager side as a fault, if you don't set it up right you're a silo. Data lives in its own world, you send tickets to the engineers to add things here or you get tickets in from other teams, that's a bad thing if that happens because as part of the office politics type of things, if people don't understand what data is doing and there's no visibility into that then, well, that effects things like budgets and promotions.
Eric Dodds (46:27)
Very true.
Max Werner (46:27)
And of course by definition you're reactive if you're a team that has to wait for other requests to come. The benefit of being in the embedded team, you know what's going on in that team, if you're the data engineering team, you have to wait and see what's coming in. And of course you tend to be more of the cost center side, depending, if you're a very, very large org. the data team makes a lot of money for the org., but on the SMB side of things if you're on data team you tend to be on the cost center which has some implications. I need to buy this tool. Well, that costs money, that's not on budget. But it helps you do the job better. It becomes a bit more of a difficult conversation.
Eric Dodds (47:13)
It is interesting. And we have some time to get to a couple of questions here, which will be great. So please raise your hand, I can unmute you or write your questions into the chat. Looks like we already had a question come in. I'll make one comment before we jump into the questions. It is interesting to see the structure of different companies. So we've seen it, some of our customers have talked about structured embedding where you sort of have centralized management of a data team but operationally the data engineers will sit on those teams as apposed to sort of shared services model where everyone comes and makes requests of sort of a centralized team and I think both can work really will. I think to your point that it depends on how you run it [crosstalk 00:48:04]-
Max Werner (48:04)
Yeah, and it's not a binary thing [crosstalk 00:48:04]-
Eric Dodds (48:04)
... and it depends on the company.
Max Werner (48:06)
... for either one structure or the other. This can be a fluid thing where, like you said, you can have a centralized data team but the people aren't embedded in other teams, but you have this one person that mostly deals with product and you have this one person that mostly deals with sales and so there is a range there as to how hard you go into one of those or two of those things.
Eric Dodds (48:30)
Sure. I'm going to read this question, it's a great one from Brain. You showed a trend where going from start-up to SMB to enterprise a company tends to go from many dispersed and varied data engineers throughout the company down to a single dedicated specialized team. We are an SMB with a dedicated data engineering team, but we're also starting our own implementation of the data mesh approach which tries to decentralize data ownership and data engineering expertise. It seems to go against the trend of your bigger company more centralized data. What are your thoughts on how data mesh changes the role of Plumber, Planner data engineers on their own team? What a great question Brian.
Max Werner (49:11)
That is a fantastic question.
Eric Dodds (49:11)
All right, Max.
Max Werner (49:14)
No, that's absolutely okay. And if you have this kind of company hang onto it for dear life because that's a great approach to have. One of the reasons that centralized data team especially on the enterprise side tend to exist like that is because other teams the data consumers have less technical knowledge, some of them might not even understand when you say, oh, this is events stream, this captures event data that has properties in it. Some of them might not understand what that means because they have their day job and they spend their 40 plus hours a week on specifically what they're doing. So you need to have that centralized team to support those people and have that English to nerd translation.
If you can get to a data mesh or data democratization approach, that's great. I've seen it rarely either tried or even successfully implemented, but if you have everybody knowing a lot more about the ABCs of data and even some people that are not on the data side but have SQL knowledge, that's great because the more self serve these people can do the overall more efficient the entire org. becomes because it's just lag time. If you don't have to go out to ask especially with data persons something or you don't have to wait until data engineer helps write a script for something, if you can do a little of that yourself even if you're a sales person, that's fantastic. So it's not that necessarily that if you are a large company that you have to have the centralized teams, that's just because very large companies tend to be a little on the older side as far as they just exists for 20, 30 plus years, that's the natural structure of less data savvy people. I hope that answers the questions.
Eric Dodds (51:23)
I think also such a good question. I'll just give a quick response here and please share additional question if anyone else has some questions. But Brian, I would say I think you're in a really great place. As an SMB I think you can conceptualize of the data mesh approach and sort of embedded data engineers from the beginning. I think as we think about larger companies there are actually some technical limitations that have led to that team structure. So I think looking back one of them is it sounds funny to say this, but it's really not until the last couple of years that it got way, way easier to actually integrate the stack. There's sort of pipeline tooling that's come of age in the last five years has actually enabled new team structures in data engineering and really very excitingly new ways of building the stack with the data mesh approach, which is really cool.
So if you think about a lot of enterprise companies who had preexisting infrastructure it's really hard for them to decentralize from literally a technical standpoint, so that's one. The other I would say and this again is sort of technical but also cultural I would say inside of a company is data governance. So the big problem with decentralization that a lot of companies have experienced is that you start to have drift in the quality of your data and so centralization in order to enforce data governance. And as you become larger your stack becomes more complex, the needs of downstream teams become more complex, sort of the reaction can be just centralize in order to force data governance.
But again, there's some technology coming out that's really exciting around how to solve that. I mean, I know at RudderStack we're building APIs that allow you to sort of run data governance on all of your pipelines, which is really cool and I mean that's sort of integrated into your own workflow and so things like that I think we'll increasingly see as the foundation for enabling that data mesh approach where people can be embedded without necessarily having the integrations or data governance problems. Really good question.
Max Werner (53:53)
Exactly to your point. It's relatively speaking new tech. I mean, Warner for instance has a system for games that collects data, sends it to a system that then does some transformation and puts it in a standardizes schema into a warehouse. Does that sound familiar as far as Rudder [inaudible 00:54:15] destination? They built that themselves from scratch because they have been doing that for a lot longer than things like no segments, no plague Rudder have been around.
Eric Dodds (54:27)
Really good question. We're out of time here. Really appreciate everyone joining. Feel free to shoot us an email if you have any questions. My email is eric@rudderstack.com. And Max your email is?
Max Werner (54:44)
My email, it's mwerner@obsessiveanalytics.com.
Eric Dodds (54:46)
Cool. Thank again for joining us. We'll follow up with an email with the recording of this, so feel free to share it and we'll catch you on the next one.
Max Werner (54:56)
See you then.