Interview: What is a CDP for Developers?
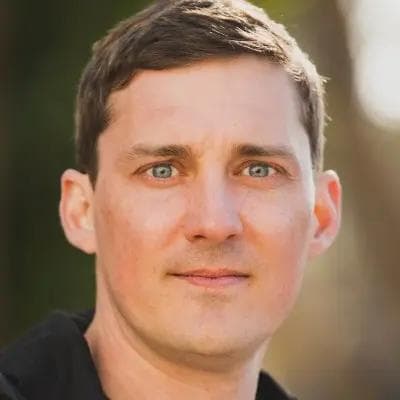
Eric Dodds
Senior Director of Product Strategy
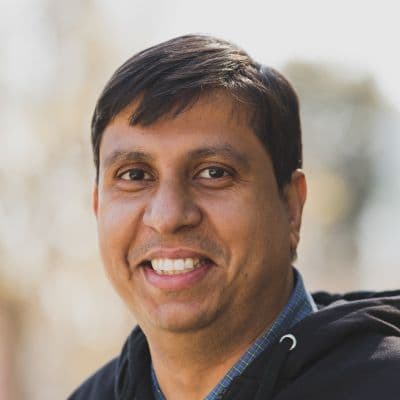
Soumyadeb Mitra
Founder and CEO of RudderStack
In this webinar, Eric will interview our founder and CEO, Soumyadeb Mitra, who will dig into the details of what a CDP for developers is and discuss why RudderStack is building developer-first tooling for customer data. At the end, he will also explain our mission and how it will grow with our recent Series A funding led by Kleiner Perkins.
- CDPs were built for marketers, but engineering needs to own the data stack
- Open source and warehouse-first - building for transparency and ownership in customer data
- Programmable pipelines - why dynamic customer data and ever-evolving data stacks require flexible, programmable pipelines
- DevOps for CDP - why customer data tooling needs to be integrated into existing developer workflows
- What’s next? The RudderStack platform as API first, the future of building on top of RudderStack pipelines
Eric Dodds (00:03)
Thank you everyone for joining us. And we have a very special guest, our founder, and CEO, Soumyadeb on the webinar. Welcome, Soumyadeb.
Soumyadeb Mitra (00:15)
Thanks, Eric. Glad to be here doing this with you.
Eric Dodds (00:21)
Great. Well, today, we're going to talk about what a CDP for developers is. A little background on this. So last week we announced that we raised a Series A from Kleiner Perkins, which is a huge milestone for our company. S28 Capital and Uncorrelated Ventures also participated. And we announced that what we're building is what we're calling a CDP for developers. And so we're going to talk about lots of different things, including the history of CDPs, and then we're going to end with the future of what we're building at RudderStack and lots of things in between.
Here's a brief agenda of what we'll cover just so you can keep in mind what we're going to chat about. And I started thinking of some questions. So we'll talk about the history of CDPs. We'll also talk about really what's the goal of using customer data and how it's changed from a marketing use case to optimizing all parts of the business. And then a particular passion of ours is the subject of why engineering needs to own the data stack which is a controversial topic, but one that we love to talk about and one that Soumyadeb has extensive experience in.
Now, we'll dig into what a CDP for developers is. We'll talk about two really key components of RudderStack, which is why we're open source and we're warehouse-first. We'll talk about what warehouse-first means in the context of a CDP. We'll run through some key developer focus features, and then Soumyadeb's going to tell us what's coming up for RudderStack as we look at the next couple of years. So without further ado, Soumyadeb, do you want to give us just a brief background on yourself, and what led you to founding RudderStack a little over two years ago?
Soumyadeb Mitra (02:08)
Yeah. Sure, Eric. So we started RudderStack in 2019 as Eric said, but I have been working in this broad space for almost eight years. Prior to RudderStack, I spent a year in a company called 8x8. And what I was mostly doing 8x8 was to build a stack, very similar to RudderStack. We are trying to pull out the customer data, building machine learning models on top for various business use cases, all the way from lead scoring, to churn prediction, to upsell prediction, and so on.
And some of the experiences doing that led me to start RudderStack. There was no tool that could really satisfy the requirements at 8x8, and it made sense to start RudderStack. Back to 8x8, I spent five years doing a startup in the B2B marketing space. I'll not get into the details, but one thing I really learned there were marketing teams only have a limited view of customer data, which makes sense because they don't control all the properties through which enterprises interact with customers.
And that is the reason we strongly believe ... And that is a hypothesis I developed in my previous company that engineering needs to own this, as we'll talk later about details. So that's my high-level background.
Eric Dodds (03:34)
Great. And I'm Eric Dodds and I run growth at RudderStack, but this is about Soumyadeb. So I won't give my background. We'll get into that a little bit actually in this section. So let's talk about a brief history of the customer data platform. So I can speak to this a little bit. So my background is actually in marketing. So I started my career in marketing. And really, it's funny looking at this chart, thinking back to 2011. I remember being a digital marketer in 2011, and I remember just being really excited about the software.
And then as subsequent years passed, the software seemed to keep getting better and better and to do more things and more things. But the acceleration's pretty crazy to go from a couple of hundred tools to 7,000 tools in less than a decade is pretty insane. And so there was almost a whiplash in the industry where the options are almost overwhelming and you struggled even to figure out which tool to pick for the job.Â
But there's a really specific reason why this dynamic happened in the MarTech landscape with marketing tools specifically. And I'll speak to this a little bit and then would love to hear your perspective on it, Soumyadeb. Really, marketing was the tip of the iceberg in terms of using customer data, right? So marketing teams in order to optimize campaigns, as they were able to collect more first-party data, use third-party data, wanted to optimize the top of the funnel to drive traffic, understand user behavior and the websites and apps, et cetera. And then also orchestrate the customer journey.
And in order to do that, really the first breed was all-in-one tools that did everything, even from your website to your email campaigns, everything lived in one place. But that created systems that were good at a number of things, but not excellent at a specific part of the puzzle. And that created point solutions. So companies that really were excellent at email marketing, but they also created data silos. They were really good at one thing, but they created a data silo, which made it hard to share the data with other teams and other tools.
And that led to an integrated MarTech stack where people were using best-of-breed tools that talk to each other. So this is the more modern generation of Salesforce and Pardot, and integrating HubSpot and Salesforce, et cetera, where your tools are talking to each other in a very integrated way. But even still, all of your data lived in different parts of the tech stack. Even though they could talk to each other, you didn't have a unified data layer.
So Soumyadeb, talk us through the integrated MarTech stack with best-of-breed actually led to needing data layer tooling and created a lot of confusion in the marketplace. Has that happened?
Soumyadeb Mitra (06:51)
This was really an interesting journey as you were saying that MarTech took from point solutions, everything in one bucket, to all these different tools. And the challenge with that approach though, as you pointed out rightly, is how do you get your data in all those tools? You have one tool for emailing, maybe another tool for your newsletters, one other tool for your push notification, one for your CRM, one for some other marketing, and so on.
You have seven tools that need to have customer data. Also, there is some feedback. So let's say you sent an email and you got a response back, and based on that response you want to take some other action. You want to send a push notification, and so on. So, that became a real mess. You've got point solutions that are very good at each task, but how do you make sure firstly, that your customer data is synced to all of them? Every time a record is created or somebody signs up, you have to sync that customer record to Salesforce and your Marketo and your push notification tool and so on.
So this was never an easy task. What people did was they built points or integrations, like one SDK for your Salesforce, some other form for Marketo, and so on. And then your website did not load properly, and that's why the record made it to Salesforce, but it never made it to Marketo. All these weird problems happen because of this silo of best-of-breed tools. And that is the problem that Segments and the Tealium’s of the world try to solve.
They said, "There is this confusion. Getting data across to all of them consistently is a problem. So just send it to us and then we'll make sure that everything goes to all the tools properly." So they've definitely added a lot of value. And that's why you see looking at the Segment's growth and trajectory, and eventual acquisition. And same for Tealium, I guess. A similar solution mostly focused on enterprises, but they're doing very well.
Eric Dodds (09:04)
And on top of that, and really at the same time, I think you saw a proliferation of CDPs that fall more on unified customer profiles and then customer journey orchestration, right? So the ActionIQs, BlueConics, et cetera. And it's funny because even though they fall under the same CDP category, they're really less about the data collection and more about actually actioning on the data. So making sure that you have really robust customer profiles and then being able to do all sorts of automation. Actually orchestrating the customer journey where people are getting emails and messages and push notifications and all that stuff.
But that led to a lot of confusion in the marketplace. And so it's pretty common for ... You see blog posts around CDPs and CDPs for all different purposes, et cetera. And of course, we are in that space as well, which we'll talk about more. But one thing I wanted to talk through was despite the confusion, the goal of what companies are trying to do with the data layer and with the activation tools is really the same. And every business is trying to optimize across business functions with customer data.
So we talked about marketing being the tip of the iceberg when it came to optimizing their function with customer data. And now we're seeing every single team across the organization, including finance have a huge hunger for customer data and first-party data because they need to optimize. Do you want to talk a little bit about that, Soumyadeb?
Soumyadeb Mitra (10:51)
Yeah, that's a great point. And firsthand saw that at 8x8. And for people who don't know 8x8, it's one of the largest telecom providers, a public company. And they're data silos. We had a mobile app which was generating a lot of ... Our customers are very using to make phone calls and text messages and so on. So there was a lot of customer data being generated in our mobile app. Then we had our billing system and we had our own homegrown CRM system and so on.
So we had multiple sources of data, and each function wanted to get access to that data. So of course, marketing, they wanted to customized journeys based on what people were doing in the apps. So that made sense. And a lot of the CDPs were trying to solve that problem. How do you define customer journeys and hear that single customer view? But the use case of customer data was well beyond marketing, as we learned at 8x8.
The next big was the use case of support. We had over 100,000 customers using our phone systems and support really wanted to know which customers are likely to churn? Which are the right customers? They used a tool called Gainsight. Gainsight is a great offer tool, but the biggest missing block was we did not have any integration from our sources of data, which was all over the place, as I mentioned, from the events springing from the app to our billing system, to our backend system, into the support Gainsight tool.
And this was important. Because let's say, what is support interested in? Who are the customers we're going to churn? Now, we found that the best predictor of churn is somebody's own usage going down over time, sending less messages, less specs, and so on. So that data comes from the average sale. But at the same time, another great feature for predicting who will churn or not is our ticketing data, which was on Sales Cloud. So bringing everything into Gainsight was very important so that the customer success team now has a view of which customers are going to churn?
So that integration was missing, and they really wanted that customer data. Now, if you go beyond support to the product. Of course, the product wanted to know who is using the product, who is not. But finance was another great example. Pricing the product was a constant degrading the company. It's always a problem in every company, of all states to public companies like 8x8. Are we rightly pricing the product? We had a free tier which was big being used. We tried up to some core limits. And then there was an unlimited tier and so on.
So how do you find out if you're pricing the product correctly? The best way to do that is to understand at each tier, what are we charging for and how are they using the product? But to do that, you need to have product data coming in, the usage data. How many calls, how many texts, and so on. So that integration was very important. Getting that data into a warehouse so that our finance team could now build out those models.
So this is just another example to highlight that the use cases of customer data are not just about marketing and sending emails. It impacts every function in the business.
Eric Dodds (14:23)
And one thing you have talked about in that experience is that you shopped around for a bunch of different tools ... You shopped a bunch of different tools to see if you could find a solution to the problem and had the realization that when you think about a CDP, it's really not a single vendor. It's actually a collection of tools that are both internal and external that you have to build into a complete customer data stack.
Soumyadeb Mitra (14:51)
100%. In fact, since this is a public thing, I don't think I should discuss much more about what tool we ended up at 8x8. But one thing I learned during that process of evaluating vendors to bring all that customer data was there is no single solution that could do that. And what I mean by that, just to reiterate what I mentioned before is your customer data is all over the place. It is being generated in your mobile app, from where people are making the phone calls and so on.
It is being generated in your backend billing system. It is there in your ticketing system. And some of it is on SaaS, some of it is in your database, in your homegrown data center, and so on. So your customer data is all over the place, and bringing everything together into one place to do all these use cases that I talked about, there was no single vendor which could do that properly. Just bringing the data together is what I'm talking about.
And then the second piece of that was once the data is in and you build some of these use cases. A good example is let's say I build a churn prediction use case, what I was talking about. Based on all this data, you build some model to predict who are the customers who are going to churn? Whatever churn the score needs to be synced back into Gainsight. So it's not just about pulling the data into a place, but also taking that output and sending it to the different tools in there.
And so there are a bunch of vendors who had excellent point solutions, one for the event stream, one for this database pulling, one for cloud pulling, one for the reverse part. But there was no single vendor. Buying four different vendors, they may not be talking to each other, they may have different formats was a big mess.
Eric Dodds (16:47)
Sure. So that's why at RudderStack, one of our core beliefs is that engineering needs to own the CDP. So tell us why that is. CDP traditionally has been a marketing term, but we're pretty convinced that modern companies, and really we see this with our customers, engineering is increasingly owning the customer data stack.
Soumyadeb Mitra (17:15)
I find it funny that the term CDP has been taken with the marketing, and the vendors who talk about CDP are only selling to marketing. If we step back and think about customer data and so on, again, going back to the use case that I was talking about, what exactly is CDP supposed to do? A customer data platform. If you didn't know anything about CDP, and we heard this term, a customer data platform. What would you expect it to do?
It should bring all the customer data into one single place. It should help you collect all the data and then it should empower you to build interesting use cases on top of that data, whether it's analytics, whether it's machine learning, whether it's simple audience creation, and so on. And then it should enable you to activate that data. Take that output and send it to all these different systems where you want that data to be sent.
So that's how I think about CDP if you've heard for the first time. And as I was saying, the use cases for CDP are all over the place. It's not just marketing, but support and finance and every function of the business. Now, going back to your question around, why should the engineering team own the CDP? If you look at all these different sources we are talking about, whether it's the mobile apps, whether it's the website, whether it's the app, whether it's the backend database, whether it's the cloud database, who has access to all these systems? Who is responsible for managing all these systems?
The marketing team doesn't. They don't care about your backend billing system. They don't even control a lot of these systems. They have no visibility or power over these mobile apps and the web apps. This is controlled by the engineering team. So if you look at it, who is in the best position to at least build integrations and figure out how to bring that data? It is the engineering team, whether it's the IT, engineering. You can argue about that, but it cannot be marketing, it cannot be financed. It has to be an engineering and some function of that. So it was almost like a no-brainer that the engineering team has to own the CDP.
Eric Dodds (19:33)
Absolutely. So engineering needs to own the CDP. And at RudderStack, we're building a CDP for developers. So talk through what a CDP for developers actually is. This is just a high-level definition that we pulled together, but we'd just love to hear in your own words, Soumyadeb, what is the CDP for developers?
Soumyadeb Mitra (19:58)
It's funny that we have to qualify that it is a CDP for developers. In my mind, CDP should be built by developers. There should be a special category of CDP for marketing, and maybe there should be some vendors in that. If we talk about platforms, CDP should be for developers. But anyway, since you don't control the category and other great people have defined this, we have to define, what is a CDP for developers?
So, we don't have [inaudible 00:20:26]. We've laid it out here, but the core idea is a platform that enables developers to bring all this customer data together and build all these interesting use cases that I talked about, all the way from analytics to machine learning. And then take that output and send it to all the downstream consumers of the data, whether it's marketing and so on. So that's how we think about a CDP or a CDP for developers.
Eric Dodds (20:52)
And as a developer yourself, why is it important for you ... And I see this in our product roadmap and just what we've built already. Why is it important for you for the CDP workflow to integrate with existing dev workflows? Because in a lot of cases ... But I would say in most cases when you think about CDP options in the marketplace, you're logging into a UI and you're clicking around a UI to execute a lot of the actions that you do as you interact with the tool.
Soumyadeb Mitra (21:29)
That's a great question. So it boils down to what does a developer does? How does a developer work? To answer that question, let's take a concrete example. Let's say I'm trying to build a lead scoring model. That's for the sake of it. And then I have this platform which is pulling all the data from your apps, and it's also pulling your CRM data and all the other sources of data into some single place, whether it's a data warehouse or data lake, doesn't matter. Some single place. And then I have this awesome lead scoring model that is deployed on top of that data.
And then it predicts a score, and then that score has to be sent back to some kind of a CRM system. Ignore the CDP part. If I'm building an end-to-end system like that, it's not easy to build and deploy and maintain and to keep that system running. This is like data pipelines. I've been a data engineer all my life. So data engineers have figured out a way to run these pipelines and version control and a release process.Â
So there is a process that we have to go through to learn these applications reliably and in a stable manner. To give you another concrete example. When you're running a data pipeline, you want that pipeline to be integrated with your paging system so that if something is broken, you want a page call or a page activity or whatever, that something is broken. As a developer, I'm used to monitoring my infrastructure in tools like Grafana or Datadog and so on top just see how things are working. Those are the tools I'm used to.
So your customer data pipeline and the applications developed on top cannot be any different. It's very important that it works with all the tools, whether it's ... Even Git. If I'm committing code and that platform is running some code for me, I want that to be integrated with my Git workflow so that I can do code reviews and all this stuff. So API force, integration with Git, all these paging integrations, become so much important when a developer is managing it right.
Eric Dodds (23:52)
Absolutely. Hey, let's talk about being open-source and being warehouse-first. And we'll start with open source. So why open source? There are lots of different ways to launch a tech company, but you chose to launch as an open-source repo on GitHub.
Soumyadeb Mitra (24:17)
That's a great question. And unlike a lot of open source projects, we started a company with open source. Now, there are multiple reasons for that. We have the high-level bullets for this, but the core thing is I don't believe in today's world you can really build a long-lasting, strong company selling to developers without being open source. Because as a developer, if I'm not a founder, and if I was looking at two solutions, one which is open source and one which is not, I'll always go with open source. This current generation of developers, we always grew up trying open source.
If I have to try a database, the number one option will be my SQL, Postgres, Mongo, whatever. I'll not go and buy Oracle to build an application. So it's just that developers love open source. And as a company, they make very natural sense that any company trying to sell to developers, they have to be open-source, I strongly believe. So that was one of the core reasons. That's the core value. Beyond that, there are more business reasons also to be open source, as we have done all these enterprise needs, all the way from the small companies to really large enterprises.
Business is also value open source. It's not just the individual developer, but the execs also value open source for all different reasons, like transparency. They want to make sure that whatever is running in their infrastructure, they have complete access to the code. It's not opening any backboards and Trojan horses and so on. So non-open source is not even a start. So this open-source really works for that kind of use case.
No vendor lock-in. This is a very common problem with SaaS companies in general. If you start using some product and you really get hooked into it, but then they jack up the prices 10X. This happened with our biggest competitor. Everybody is upset, but they cannot move out. Now, because we are open source, we have a natural competition. If we do that, a lot of our customers will switch to the open-source product, which is equally performing.
Is it bad for the company? I don't think so, because this helps us to always be honest about the value we are delivering to the company and not get customers hooked and jack up the prices later. And definitely for the buyer, this makes total sense. This gives them more level ... Finally, community. A lot of the open-source projects are built on community. Community contribution is extremely important. That's how the open-source project gets started. Because we are a VC-funded company it was less of an issue because we had engineers who are working on it, but where it really helped for us was our integration.
So we're finally integrating with tens and hundreds of tools, and the community has been very helpful in giving us feedback, fixing issues on those integrations, which are very hard to find out, because we just don't have that much engineering power.
Eric Dodds (27:57)
Sure. And I would say, even though I haven't been here since the beginning. I came in when we were still very small, especially early from a product perspective. In Maine, we've had some really good ideas come from the community that has turned into key features, which has been just a really neat thing to see that you ... Of course, you can always get customer feedback, but it's such a different dynamic when you're helping someone with an open-source tool and you're solving a really key problem for them, and then they're willing to give back to you. It's just a really new dynamic.
Warehouse-first. So one really unique thing that is such a drastic differentiator between RudderStack and a lot of the other CDPs and even data infrastructure tools out there is that we don't store any data. And you communicate that as a warehouse-first mindset when it comes to the product and really even the whole customer data stack. So talk us through why you made the decision to build the product as a warehouse-first product that doesn't store data.
Soumyadeb Mitra (29:12)
This is one of those things of CDP for developers. It's unfortunate that we have to highlight that we are warehouse-first. Because as an engineer, if I'm pulling through the customer data stack, the kind of use cases I talked about, bring customer data into someplace and do interesting things with that data and then activate that data. If an engineer is building that stack, they have to build it around some data store.
You cannot do it on completely a third-party SaaS where they have complete access to the data, and then you only get an API to look up specific profiles. How can you build applications on top? How can you run SQL queries? How can you train your machine learning models if all your data is with a third-party vendor that you only have API access to? You cannot do any interesting use case on top of the data.
So it's almost no-brainer that a customer data platform should run on top of some data store, whether it's a warehouse, whether it's a data lake, whether it's even a database like Postgres, but it has to be on top of some database. And that's how it should be architected. But unfortunately, every other CDP is architected the other way. Maybe it makes sense for them when they're seeling to the marketers and they don't want to control the data warehouse and they don't want to worry about the database and so on, which makes sense. I understand that. But when an engineer is trying to build the next generation CDP, it has to be on some kind of a store that you control.
So that's the baseline. Of course, we enable that. And by not storing any data and giving the entire data to our customers, you also get some additional benefits, like data ownership and privacy, and security. With all these GDPR and CCPA regulations, there is a very big focus on not sending data to third-party vendors. More and more enterprises are getting careful about what data is being shared with whom and so on.
So this is the other benefit of not storing data in a warehouse-first architecture where the warehouse is controlled by the customer. And then I already talked about the last point where once you have access to the data, then you can create your machine learning model, you can run SQL queries, you can go crazy. That is not possible if your data is just available through narrow APIs.
Eric Dodds (31:58)
True. And I would say that really in the last several years, this has become even more exciting, because of how much advancement there's been in the warehouse space. So now you have all sorts of interesting machine learning as a service type functionality happening on the warehouses, and people are literally building applications on top of data warehouses, which sounds crazy, but the architectures and tooling around that have become incredible. And so I think we're going to see some really, really interesting things in the next five years as that technology becomes even more advanced.
Soumyadeb Mitra (32:41)
That's a great point. In fact, I'd just like to highlight one of our customer's use cases. So they are dumping all the customer data into BigQuery. And BigQuery has this BigQuery ML, where you can train a machine learning model on top of BigQuery using just SQL. You don't have to learn Python modules or anything. Just a simple SQL query lets you print a model. And they built some kind of a churn prediction score just with BigQuery SQL. And they're using that score to send out free coupons to bring ... They're highly likely to churn customers.
And this end-to-end stack built with just RudderStack and BigQuery increase their revenue by 10%. So that was amazing, looking at the minimal effort they had to put. They didn't have any data scientists. They had a couple of engineers who put together this end-to-end use case. Again, you cannot build these use cases if you don't have access to the data and the data is not in a warehouse-like BigQuery.
Eric Dodds (33:46)
Right. It's really exciting. I think one dynamic we talk about a lot is that I think one of the really exciting things for engineering teams and data engineers and people working on the stack is that we've spent a really long time, out of necessity, on low-level plumbing problems from a technical perspective, just because it's been really hard to get these things working. As the technology has advanced though, now people are starting to work on things that are way more interesting and way more valuable because the low-level plumbing problems and the available features like the ML stuff and BigQuery are allowing smaller teams to do way more than they ever could afford, which is really exciting.
Soumyadeb Mitra (34:33)
The other thing I'll just add here is I think the warehouses themselves have become so much more accessible. 10 years back or 12 years back before that shift, you have to go and buy Teradata and other warehouse tools. Now you can spin up a warehouse in two minutes and pay $100 a month and have a real warehouse. You can scale up to terabytes. So that was another big thing that is driving these use cases.
Eric Dodds (35:00)
Absolutely. The cost of storing and running queries on data is certainly decreasing, which is really exciting. Let's talk through a few key features here in terms of the problems that RudderStack solves as a CDP for developers. So this one seems obvious, but integration is a really big challenge when you're building a customer data stack. And one thing I want to talk about specifically. Of course, you want to send data to downstream marketing tools and sales tools, and product analytics tools, but I think one of the things that are really interesting in the way that we've seen customers use RudderStack is integrating with core infrastructure tools.
So in this screenshot, you can see Redis there, but we also support Kafka, we support Kinesis. We really support a lot of things that are core pieces of internal components of data tooling. Do you want to talk a little bit about that and maybe why that was really important to you as far as our roadmap?
Soumyadeb Mitra (36:06)
Yeah. I think it again goes back to the CDP for the developer’s use case. And maybe I'll give a concrete use case. Getting the customer data is interesting, dumping into a warehouse, building reports, SQL models, and the machine learning models, all that is exciting. But one of the big use cases is personalization, where you want to collect all this data and then drive your app personalization, whether it's in-app recommendations or email personalization, where next email to send or products to promote through RudderStack.
So for the app personalization use case, what do you need? You need some kind of a profile store where you store your customer reports. And then you want to update that profile store with recommendations. And then you want to consume that profile store in your app through an API. And that's how you want to personalize your app. So to do that, you need that high-performance key-value store, something like Redis or Cassandra or any other key-value store that you can look up from your app. But then the profile has to be created by someone.
The profile has to be enhanced with recommendations from your machine learning model. So that pipeline becomes pretty complicated. You have to send your event stream to somewhere, train your machine learning models, take the outputs, sync it back to the profile store, initially create the profiles in that tool. So this pipeline gets pretty messy pretty fast. And this is what RudderStack really simplifies.Â
You can literally connect RudderStack to Redis, and RudderStack will create those user profiles in Redis. Then you can send that data into a warehouse. You can build your machine learning models using BigQuery ML or whatever you like. And then you come up with some recommendations. And those recommendations, you want to sync in back to Redis. Again, instead of you having to run the pipeline, RudderStack can do that.
It will put that data in sync, and now you have a rich profile in Redis with the user profile and all the recommendations that you can now consume in your app. So again, this may be a complicated architecture to explain in words, but these are the use cases that are made really simple by RudderStack because of our integration. Because again, back to our CDP for developers, we're not just enabling marketing. We are enabling every other function, of course, including the engineering themselves. So those things are very important.
There are similar use cases for Kafka and so on. I don't want to get into the details, but we have some content around that.
Eric Dodds (38:47)
Sure. I was talking with one of our customers in the e-commerce space. He's actually using the Redis personalization architecture. And they made a really interesting point in that in e-commerce you have low-level out-of-the-box recommendations engines that have a one-size-fits-all algorithm that maybe you can do some light customization on. But the gap between that and then actually delivering personalization with your own model is so big that it's almost impossible for small companies to build because it requires so much of those low-level plumbing challenges in terms of engineering work.
All right. Transformations. This is probably our most loved feature, and it can do so many different things. And actually, I don't think I know the story. Where did the idea for transformations come from? And it's been around since the beginning, but it's become incredibly powerful at this point.
Soumyadeb Mitra (39:56)
This also came from one of the use cases that we were trying to build at 8x8. It's a very simple use case. Every time there is a support request, whether it's a chat or an email that is sent to our support tool, which was Salesforce, pull that data out, do some simple machine learning sentiment analysis. And if it is a really upset customer and the sentiment is really bad, send a notification somewhere. In that case, we wanted to send it to Gainsight, but you could think of sending it to Slack or whatever.
So an end-to-end use case where you are getting an event, doing some transformations on it, which is calling an API to figure out that sentiment and then sending it out. So it, again, made sense that when you are building this pipeline of collecting data and then sending it out, you should have some ability to write transformation code on that track. So it was really that use case which we thought it makes sense to do it. But then over time, we learned that there are so many different use cases of transformation, all the way from simple fixing events.
You ship your mobile app and you made some errors. Some event name is spelled wrong, and it's different from what was before and you want to fix it. Shipping a new version is hard, so you can just write a transformation. It's like a JavaScript function that you run and that runs on the backend. So the event comes in, the transformation fixes it, and then it goes to your warehouse that goes wherever. So that is a very, very commonly used use case.
There are other use cases like PII scanning. You're getting all these events, but you're really not sure if your engineer is embedding PII accidentally or whatever. But then there are regulations around it not sending PII to your warehouse or some other cloud tools. So you can write a transformation in reference to the PII. The example that we have here, which we use internally is around Clearbit. Somebody signs up on the website, we want to send that record to Salesforce, but then we want to call Clearbit and enhance that record with additional fields before it hits Salesforce.
Again, write a transformation that calls that cleared with API and does that. Again, it came from a very simple use case that we thought we should enable, but then over time ... I didn't even expect it to be so popular, but every customer we have is running some transformation.
Eric Dodds (42:24)
And I think when you think about the PII use case, what makes it so interesting and so powerful is that we don't store any data. So there's no data persistence. So when you remove the PII from a security and privacy standpoint, it just makes so much sense. API-based data governance. Now, we have lots of features that we're working on around this that we won't talk about quite yet. But I think what I'd love to talk about is data governance is a huge topic. It's very complex in the modern data stack.
And there are a lot of interesting solutions out there that are trying to solve this from a team collaboration UI standpoint, but you made the decision to approach this API first, which makes sense from appealing to developers and building for developers, but explain that decision a little bit more and the thought behind it.
Soumyadeb Mitra (43:21)
This is again something we learned from our customers. The core problem that we are trying to solve is you have all these hundreds of events being sent to RudderStack, some coming from their mobile apps, some from the website, and so on. And particularly if you're a large org, you may have different teams sending events to your backend and those events are being sent to different places. And then we want some quality control.
For example, in your QA life cycle, you want to make sure that all the events are conforming to some standard. It could be as simple as they don't have PII, to as complex as these are the keys, these are the values. All the required keys are present and so on. Now, if you want to build that use case, and if you have hundreds of events generated from different teams, how else would you do it? If you build a UI that shows that, okay, now you have a violation, who goes to the UI and checks that this event is bad, this event is good? You cannot scale that with just a UI.
On the other hand, if you have an API that you can query, you can integrate that into your CI/CD pipelines, your nightly tests, whatever. So the API first approach makes so much sense when talking to developers. It's a no-brainer, but again, this was learning that we had when we spoke to our customers.
Eric Dodds (44:53)
Sure. And then we already talked about this a little bit, but talk about dev-friendly monitoring and alerting. So Grafana dashboards, integrations with Datadog. Those are hugely popular features.
Soumyadeb Mitra (45:12)
And it goes back to my previous point. If I'm managing this data pipeline, like a customer data pipeline and some application on top, this is not the only thing I'm doing. I am responsible for three other pipelines and some of them are internal pipelines and so on. And I probably have some kind of a monitoring tool to make sure everything is working. So it's either a Grafana. Grafana is hugely popular in developers and so on, or it could be some paid solution like Datadog or New Relic or something. There are a bunch of variants.
So it's important that I have a single dashboard to do that. I don't want to go to five different dashboards to check the status of my pipeline. So again, very obvious thing when you're building for developers. But again, a lot of our competition doesn't do that because they are not selling to developers. So they are building for the marketing personnel. The marketing people don't come to Grafana. So they would rather have that data in one integrated UI as opposed to the tools like Grafana.
Eric Dodds (46:21)
Sure. Well, we're coming up close to time here. I want to leave a few minutes for questions. But give us a quick couple of minutes on what we're building next at RudderStack.
Soumyadeb Mitra (46:36)
So there are multiple ways to answer this question. One is what is immediate in the next quarter? And I think the big focus for us is two things, make sure that our pipelines are really mature. Lots of integrations to build both on the source site, as well as destination side. We are building this warehouse action, which is ... The category now it's called reverse ETL. Taking data out of your system and sending it to somewhere else, although I don't like the term reverse ETL because the use cases are all over.
You also have the use case that I talked about, where you want to pull the Zendesk chat and then in real-time, do sentiment analysis and send it back into Gainsight or Slack. Is that ETL? Is this reverse ETL, or is it a combination of both? Anyway, so there's a lot of things on the pipeline that we are working on beyond integrations. But one of the big focuses for us is also around data quality with data governance.
This, again, we have learned talking to our customers is bad events come in, that great support, what tooling can we build around solving that? Again, we can probably do an entire webinar on that, but that's the high level of what we are working on this quarter. So that's the short-term thing. The long-term vision is to almost make your data pipelines like your plumbing in your home. You should not have to worry about that. It should just work. You shouldn't even see that.
The aim is to make it really easy for you to develop these applications on top, whether it's a machine learning application or it's a BBT model without worrying about the data pipeline. Again, it may seem a bit [inaudible 00:48:23], but we have a concrete plan to execute through that vision. So hopefully we'll have more innovation in that direction.
Eric Dodds (48:30)
Very cool. Alrighty. Well, we have a couple of minutes for questions. Feel free to raise your hand or type the question into the chat, and we would be happy to have an answer. I can also unmute you if you raise your hand. And you can ask about anything. We have the founder here. So anything you want to know, I guess within reason. One question came through. What were you doing before 8x8?
Soumyadeb Mitra (49:09)
So I was the founder and CTO of this company called MarianaIQ. We were building the next-generation marketing automation system. So an AI-driven system analyzes all the customer data and recommends what is the next best action. Send an email, making calls. So that was the vision. We built parts of it. We built a lead scoring model. We built some kind of a next action recommender and so on. It's about the things we built around that vision.
I don't want to take too long to answer that, but one thing I really learned there is we sold to the marketing teams, and marketing teams really did not have a lot of data about the customers. They had some data about maybe their email opens and then website visits, but nothing beyond that. So building some of those use cases was really hard without that data. And that was the key learning where marketing teams cannot build these things. And you need to be in the engineering org to do that.
Eric Dodds (50:16)
I had another question here, which is a great question. In terms of CDPs that are on the activation side ... So the question is, for CDPs that do activation like emails, et cetera, do you compete with those CDPs, or do you work with them?
Soumyadeb Mitra (50:40)
We are partners to them. The way I think about it is like we are building a platform for developers to collect all the customer data and then do things with that and then activate that. But then there are concrete marketing use cases. There are use cases around building customer journeys and audiences and predictive audiences and so on. So there are strong marketing use cases, just like there are strong use cases for any vertical.
So for the marketing use cases, that's not our strength. We don't sell to the market because we don't understand their use cases and so on. So we partner with those vendors, whose strength is that.
Eric Dodds (51:27)
Sure. Right. I have another question here. Unless another one comes in between, we can end it after this one, because we're close to the hour. What does the Series A change for RudderStack, and what does it mean for the company going forward?
Soumyadeb Mitra (51:46)
It's a great validation of our vision and our belief. So I'm really excited about that. What it lets us do is number one, innovate more on the product and engineering side. There's so much to build before we are even close to our vision. So that will be a big focus, but also go to market is another big focus. I think there are a lot of companies that can benefit from a solution like that. So really need to accelerate and grow that.
Eric Dodds (52:17)
Great. Well, thank you, Soumyadeb. I know you are a very busy man, and I appreciate you taking the time. Thank you to everyone who joined. We'll send you a follow-up that has a link to the recording of this. And feel free to reach out if you have any questions.
Soumyadeb Mitra (52:32)
Thanks, Eric for having me. It was really great doing this.
Eric Dodds (52:36)
All right. Have a great day.